The Beginning of Moving Our Environment into the Cloud
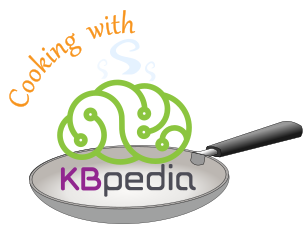
Today’s installment in our Cooking with Python and KBpedia series begins a three-part mini-series of moving our environment into the clouds. This first part begins with setting up a remote instance and Web page server using the mini-framework Flask. The next installment expands on this basis to begin adding a SPARQL endpoint to the remote instance. And, then, in the concluding third part to this mini-series, we complete the endpoint and make the SPARQL service active.
We undertake this mini-series because we want to make KBpedia more open to SPARQL queries from anywhere on the Web and we will be migrating our main KBpedia Web site content to Python. The three installments in this mini-series pave the way for us to achieve these aims.
We begin today’s installment by looking at the approach and alternatives for achieving these aims, and then we proceed to outline the steps needed to set up a Python instance on a remote (cloud) instance and to begin serving Web pages.
Approach and Alternatives
We saw earlier in CWPK #25 and CWPK #50 the usefulness of the SPARQL query language to interact with knowledge graphs. Our first objective, therefore, was to establish a similar facility for KBpedia. Though we have an alternate choice to set up some form of RESTful API to KBpedia (see further Additional Documentation below), and perhaps that may make sense at some point in time, our preference is to use SPARQL given its robustness and many query examples as earlier installments document.
Further, we can foresee that our work with Python in KBpedia may warrant our moving our standard KBpedia Web site to that away from Clojure and its current Bootstrap-based Web page format. Though Python is not generally known for its Web-page serving capabilities, some exploration of that area may indicate whether we may go in that direction or not. Lastly, given our intent to make querying of KBpedia more broadly available, we also wanted to adhere to linked data standards. This latter objective is perhaps the most problematic of our aims as we will discuss in a couple of installments.
Typically, and certainly the easiest path, when one wants to set up a SPARQL endpoint with linked data ‘conneg‘ (content negotiation) is to use an existing triple store that has these capabilities built in. We already use Virtuoso as a triple store, and there are a couple of Python installation guides already available for Virtuoso. Most of the other widely available triple stores have similar capabilities.
Were we not interested in general Web page serving and were outside of the bounds of the objectives of this CWPK series, use of a triple store is the path we would recommend. However, since our aims are different, we needed to turn our attention to Python-based solutions.
From the standpoint of Web-page serving, perhaps the best known and most widely installed Python option is Django, a fully featured, open-source Web framework. Django has a similar scope and suite of capabilities to its PHP counterpart, Drupal. These are comprehensive and complicated frameworks, well suited to massive database-backed sites or ones with e-commerce or other specialty applications. We have had much experience with Drupal, and frankly did not want to tackle the complexity of a full framework.
I was much more attracted to a simpler microframework. The two leading Python options are Flask and Bottle (though there is also Falcon, which does not seem as developed). I was frankly more impressed with the documentation and growth and acceptance shown by Flask, and there appeared to be more analogous installations. Flask is based on the Jinja template engine and the Werkzeug WSGI (Web-server Gateway Interface) utility library. It is fully based on Unicode.
Another factor that needs to be considered is support for RDFlib the key package (and related) that we will be using for the SPARQL efforts. I first discussed this package in CWPK #24, though it is featured in many installments.
Basic Installation
We will be setting up these new endpoints on our cloud server, which is a large EC2 instance on Amazon Web Services running Ubuntu 18.04 LTS. Of course, this is only one of many cloud services. As a result, I will not discuss all of the preliminary steps to first securing an instance, or setting up an SSH client to access it, nor any of the initial other start-up issues. For EC2 on AWS, there are many such tutorials. Two that I have encountered in doing the research for this installment include this one and this other one. There are multiple others, and ones applicable to other providers than AWS as well.
So, we begin from the point that an instance exists and you know how to log in and do basic steps with it. Even with this simplification, I began my considerations with these questions in mind:
- Do I need a package manager, and if so, which one?
- Where should I place my Python projects within the remote instance directory structure?
- How do I also include the pip package environment?
- Should I use virtual environments?
With regard to the first question, I was sure I wanted to maintain the conda package manager, but I was not convinced I needed the full GUI and set of packages with Anaconda. I wanted to keep consistency with the set-up environment we put in place for our local instance (see CWPK #9). However, since I had gained much experience with Anaconda and conda, I felt comfortable not installing the full 4 GB full Anaconda distribution. This led me to adopt the minimal miniconda package, which has a much smaller footprint. True, I will need to install all specific Python packages and work from the command line (terminal), but that is easy given the experience I have gained.
Second, in reviewing best practices information for directory structures on Ubuntu, I decided to create an analogous ‘python-projects’ master directory, similar to what I established for the local instance, under the standard user application directory. I thus decided and created this master directory: usr/bin/python-projects
.
So, having decided to use miniconda and knowing the master directory I wanted to use, I proceeded to begin to set up my remote installation. I followed a useful source for this process, though importantly updated all references to the current versions. The first step was to navigate to my master directory and then to download the 64-bit Linux installer onto my remove instance, followed by executing the installation script:
//repo.anaconda.com/miniconda/Miniconda3-latest-Linux-x86_64.sh * make sure you use updated version
wget https:
-latest-Linux-x86_64.sh sh Miniconda3
The installation script first requires you to page through the license agreement (many lines!!) and then accept it. Upon acceptance, the Miniconda code is installed with some skeletal stubs in a new directory under your master directory, which in my case is /usr/bin/python-projects/miniconda3
. The last step in the installation process asks where you want Miniconda installed. The default is to use root/miniconda3
. Since I wanted to keep all of my Python project stuff isolated, I overrode this suggestion and used my standard location of /usr/bin/python-projects/miniconda3
.
After all of the appropriate files are copied, I agreed to initialize Miniconda. This completes the basic Miniconda installation.
After signing back in, I again navigated to my Python master directory and then installed the pip package manager since not all of the Python packages we use in this CWPK series and cowpoke are available through conda.
Virtual Environments and a Detour
In the standard use of a Linux installation, one uses the distro’s own installation and package management procedures. In the case of Ubuntu and related distros such as Debian, apt
stands for ‘advanced package tool‘ and through commands such as apt-get
is one means to install new capabilities to your remote instance. Other Linux distros may use yum
or rpm
or similar to install new packages.
Then, of course, within Python, one has the pip
package installer and the conda
one we are using. Further, within a Linux installation, how one may install packages and where they may be applicable depends on the user rights of the installer. That is one reason why it is important to have sudo
(super user) rights as an administrator of the instance when one wants new packages to be available to all users.
These package managers may conflict in rights and, if not used properly, may act to work at cross purposes. For example, in a standard AWS EC2 instance using Ubuntu, it comes packaged with its own Python version. This default is useful for the occasional app that needs Python, but does not conform to the segregation of packages that Python often requires using its ‘virtual environments‘.
On the face of it, the use of virtual environments seems to make sense for Python: we can keep projects separate with different dependencies and even different versions of Python. I took this viewpoint at face value when I began this transition to installing Python on a remote instance.
Given this, it is important to never run sudo pip install
. We need to know where and to what our Python packages apply, and generic (Linux-type) install routines confuse Linux conventions with Python ones. We are best in being explicit. There are conditions, then, where the idea of a Python virtual environment makes sense. These circumstances include, among others, a Python shop that has many projects; multiple developers with different versions and different applications; a mix of Python applications between Python 2 and 3 or where specific version dependencies create unworking conditions; and so forth.
However, what I found in migrating to a remote instance is that virtual environments, combined with a remote instance and different operating system, added complexity that made finding and correcting problems difficult. After struggling for hours trying to get systems to work, not really knowing where the problem was occurring nor where to look for diagnostics, I learned some important things the hard way. I describe below some of these lessons.
An Unfortunate Bad Fork
So, I was convinced that a virtual environment made sense and set about following some online sources that documented this path. In general, these approaches used virtualenv
(or venv
), a pip-based environment manager, to set up this environment. Further, since I was using Ubuntu, AWS and Apache2, these aspects added to the constraints I needed to accommodate to pursue this path.
In implementing this path, I first installed pip
:
-y python3-pip sudo apt install
I was now ready to tackle the fourth and last of my installation questions, namely to provide a virtual environment for my KBpedia-related Python projects. To do so, we first need to install the virtual environment package:
-y python3-venv sudo apt install
Then, we make sure we are in the base directory where we want this virtual environment to reside. (In our case, /usr/bin/python-projects/
. We also will name our virtual environment kbpedia
. We first establish the virtual environment:
.6 -m venv kbpedia python3
which pre-populates a directory with some skeletal capabilities. Then we activate it:
/bin/activate source kbpedia
The virtual environment is now active, and you can work with it as if you were are the standard command prompt, though that prompt does change in form to something like (kbpedia) root@ip-xxx-xx-x-xx:/usr/bin/python-projects#
. You work with this directory as you normally would, adding test code next in our case. When done working with this environment, type deactivate
to return from the virtual environment.
The problem is, none of this worked for my circumstance, and likely never would. What I had neglected in taking this fork is that conda
is both a package manager and a virtual environment manager. With the steps I had just taken, I had inadvertently set up multiple virtual environments, which were definitely in conflict.
The Proper Installation
Once I realized that my choice of conda
meant I had already committed to a virtual environment manager, I needed to back off all of the installs I had undertaken with the bad fork. That meant I needed to uninstall all packages installed under that environment, remove the venv
environment manager, remove all symbolic links, and so forth. I also needed to remove all Apache2 updates I had installed for working with wsgi
. I had no confidence whatever I had installed had registered properly.
The bad fork was a costly mistake, and it took me quite of bit of time to research and find the proper commands to remove the steps I had undertaken (which I do not document here). My intent was to get back to ‘bare iron’ for my remote installation so that I could pursue a clean install based on conda
.
Installing the mod-wsgi Apache Module
After reclaiming the instance, my first step was to install the appropriate Apache2 modules to work with Python and wsgi
. I began by installing the WSGI module to Apache2:
sudo apt-get install libapache2-mod-wsgi-py3
Which we then test to see if it was indeed installed properly:
apt-cache show libapache2-mod-wsgi-py3
Which displays:
Package: libapache2-mod-wsgi-py3
Architecture: amd64
Version: 4.5.17-1ubuntu1
Priority: optional
Section: universe/httpd
Source: mod-wsgi
Origin: Ubuntu
Maintainer: Ubuntu Developers <ubuntu-devel-discuss@lists.ubuntu.com> Original-Maintainer: Debian Python Modules Team <python-modules-team@lists.alioth.debian.org> Bugs: https://bugs.launchpad.net/ubuntu/+filebug Installed-Size: 271 Provides: httpd-wsgi Depends: apache2-api-20120211, apache2-bin (>= 2.4.16), python3 (>= 3.6), python3 (<< 3.7), libc6 (>= 2.14), libpython3.6 (>= 3.6.5) Conflicts: libapache2-mod-wsgi Filename: pool/universe/m/mod-wsgi/libapache2-mod-wsgi-py3_4.5.17-1ubuntu1_amd64.deb Size: 88268 MD5sum: 540669f9c5cc6d7a9980255656dd1273 SHA1: 4130c072593fc7da07b2ff41a6eb7d8722afd9df SHA256: 6e443114d228c17f307736ee9145d6e6fcef74ff8f9ec872c645b951028f898b Homepage: http://www.modwsgi.org/ Description-en: Python 3 WSGI adapter module for Apache The mod_wsgi adapter is an Apache module that provides a WSGI (Web Server Gateway Interface, a standard interface between web server software and web applications written in Python) compliant interface for hosting Python based web applications within Apache. The adapter provides significantly better performance than using existing WSGI adapters for mod_python or CGI. . This package provides module for Python 3.X. Description-md5: 9804c7965adca269cbc58c4a8eb236d8 </python-modules-team@lists.alioth.debian.org></ubuntu-devel-discuss@lists.ubuntu.com>
And next, we check to see if the module is properly registered:
Our first test is:
sudo apachectl -t
and the second is:
sudo apachectl -M | grep wsgi
which gives us the correct answer:
wsgi_module (shared)
Setting Up the Conda Virtual Environment
We then proceed to create the ‘kbpedia’ virtual environment under conda
:
conda create -n kbpedia python=3
Which we test with the Ubuntu path inquiry:
echo $PATH
which gives us the correct path registration (the first of the five listed):
/usr/bin/python-projects/miniconda3/envs/kbpedia/bin:/usr/bin/python-projects/miniconda3/condabin:/usr/local/sbin:/usr/local/bin:/usr/sbin:/usr/bin:/sbin:/bin:/snap/bin
and then we activate the ‘kbpedia’ environment:
conda activate kbpedia
Installing Needed Packages
Now that we are active in our virtual environment, we need to install our missing packages:
conda install flask
conda install pip
Installing Needed Files
For Flask to operate, we need two files. The first file is the basic calling application, test_kbpedia.py
, that we place under the Web sites directory location, /var/www/html/kbpedia/
(we create the kbpedia
directory). We call up the editor for this new file:
vi test_kbpedia.py
and enter the following simple Python program:
from flask import Flask
app = Flask(__name__)@app.route("/")
def hello():
return "Hello KBpedia!"
It is important to know that Flask comes with its own minimal Web server, useful for only demo or test purposes, and one (because it can not be secured) should NOT be used in a production environment. Nonetheless, we can test this initial Flask specification by entering either of these two commands:
wget http://127.0.0.1/ -O-
curl http://127.0.0.1:5000
Hmmm. These commands seem not to work. Something must not be correct in our Python file format. To get better feedback, we invoke Python and then:
flask run
This gives us the standard traceback listing that we have seen previously with Python programs. We get an error message that the name ‘app’ is not recognized. As we inspect the code more closely, we can see that one line in the example that we were following was inadvertenly truncated (denoted by the decorator ‘@’ sign). We again edit test_kbpedia.py
to now appear as follows:
from flask import Flask
app = Flask(__name__)
@app.route("/")
def hello():
return "Hello KBpedia!"
Great! That seems to fix the problem.
We next need to enter a second Python program that tells WSGI where to find this app. We call up the editor again in the same directory location:
vi wsgi.py
(We also may use the *.wsgi
file extension if we so choose; some examples prefer this second option.)
and enter this simple program into our editor:
import sys
sys.path.insert(0, "/var/www/html/kbpedia/")
from test_kbpedia import app as application
This program tells WSGI where to find the application, which we register via the sys
package as the code states.
Lastly, to complete our needed chain of references, Apache2 needs instructions for where a ‘kbpedia’ reference in an incoming URI needs to point in order to find its corresponding resources. So, we go to the /etc/apache2/sites-enabled
directory and then edit this file:
vi 000-default.conf
Under DocumentRoot
in this file, enter these instructions:
WSGIDaemonProcess kbpedia python-path=/usr/bin/python-projects/miniconda3/envs/kbpedia/lib/python3.8/site-packages
WSGIScriptAlias /kbpedia /var/www/html/kbpedia/wsgi.py
<Directory /var/www/html/kbpedia>
WSGIProcessGroup kbpedia
WSGIApplicationGroup %{GLOBAL}
Order deny,allow
Allow from all
</Directory>
This provides the path for where to find the WSGI file and Python.
We check to make sure all of these files are written correctly by entering this command:
sudo apachectl -t
When we first run this, while we get a ‘Syntax OK’ message, we also get a warning message that we need to “Set the ‘ServerName’ directive globally to suppress this message”. While there is no problem to continue in this mode, in order to understand the suite of supporting files, we navigate to where the ServerName
is set in the directory /etc/apache2
by editing this file:
vi apache2.conf
and enter this name:
ServerName localhost
Note anytime we make a change to an Apache2 configuration file, that we need to re-start the server to make sure the new configuration is now active. Here is the basic command:
sudo service apache2 restart
If there is no problem, the prompt is returned after entering the command.
We are now complete with our initial inputs. To test whether we have properly set up Flask and our Web page serving, we use the IP for our instance and enter this command in a browser:
And, the browser returns a Web page stating:
Hello KBpedia!
Fantastic! We now are serving Web pages from our remote instance.
Of course, this is only the simplest of examples, and we will need to invoke templates in order to use actual HTML and style sheets. These are topics we will undertake in the next installment. But, we have achieved a useful milestone in this three-part mini-series.
Some More conda Commands
If you want to install libraries into an environment, or you want to use that environment, you have to activate the environment first by:
conda activate kbpedia
After invoking this command, we can install desired packages into the target environment, for example:
conda install package-name
But sometimes we need to use a different channel, in which case we first need to install that channel:
conda install --channel asmeurer
then, invoke it (say):
conda install -c conda-forge package-name
To see what packages are presently available to your conda environment, type:
conda list
And, to see what environments you have set up within conda
, enter:
conda env list
which, in our current circumstance, give us this result:
base /usr/bin/python-projects/miniconda3
kbpedia * /usr/bin/python-projects/miniconda3/envs/kbpedia
The environment shown with the asterisk (*
) is the currently active one.
Another useful command to know is to get full information on your currently active conda environment. To do so, type:
conda info
in our case, that produces the following output:
active environment : kbpedia active env location : /usr/bin/python-projects/miniconda3/envs/kbpedia shell level : 1 user config file : /root/.condarc populated config files : conda version : 4.8.4 conda-build version : not installed python version : 3.8.3.final.0 virtual packages : __glibc=2.27 base environment : /usr/bin/python-projects/miniconda3 (writable) channel URLs : https://repo.anaconda.com/pkgs/main/linux-64 https://repo.anaconda.com/pkgs/main/noarch https://repo.anaconda.com/pkgs/r/linux-64 https://repo.anaconda.com/pkgs/r/noarch package cache : /usr/bin/python-projects/miniconda3/pkgs /root/.conda/pkgs envs directories : /usr/bin/python-projects/miniconda3/envs /root/.conda/envs platform : linux-64 user-agent : conda/4.8.4 requests/2.24.0 CPython/3.8.3 Linux/4.15.0-115-generic ubuntu/16.04.1 glibc/2.27 UID:GID : 0:0 netrc file : None offline mode : False
Additional Documentation
Here are some additional resources touched upon in the prior discussions:
Getting Oriented
- The Definitive Guide to Conda Environments
- Deploy Flask apps using Anaconda on Ubuntu Server
- Why You Need Python Environments and How to Manage Them with Conda
- Conda Best Practices.
Flask on AWS
Each of these cover some of the first steps needed to get set up on AWS, which we skip over herein:
- Deploy on AWS is a nice starting point, but not specific to our conda + AWS + Flask + Apache2 environment
- Flask and Apache and AWS is also pretty good, but lacks conda
- Another Deploy on AWS with the later steps useful, from about #7 forward.
RESTful APIs
- https://python-rest-framework.readthedocs.io/en/latest/tutorial/first_step.html (Python REST API) (a set of utilities based on werkzeug)
- https://pypi.org/project/endpoints/
- https://programminghistorian.org/en/lessons/creating-apis-with-python-and-flask
- https://rapidapi.com/blog/how-to-use-an-api-with-python/
- https://auth0.com/blog/developing-restful-apis-with-python-and-flask/
- Flask-RESTful
- Another source includes use of Swagger UI documenter and Flask-RESTplus.
General Flask Resources
- A good, official introductory site to Flask
- Useful reference on Flask as a Web framework
- Another good starting point that also uses SQLite3 as an example backend database
- The Mega Tutorial, and there is a follow-up with emphasis on a RESTful Web API.
RDFlib
Here is a nice overview of RDFlib.
General Remote Instance Set-up
A video on setting up an EC2 instance and Putty; also deals with updating Python, Filezilla and crontab.
Other Web Page Resources
- Ordia is a Wikimedia tools app built on Flask that may have some aspects relevant to an eventual KBpedia Web site
- Converting Jupyter to WordPress HTML
- We may also want to bring some graph visualizations to our Web pages. One interesting package is Webweb and its related paper; another is WebVOWL.
*.ipynb
file. It may take a bit of time for the interactive option to load.