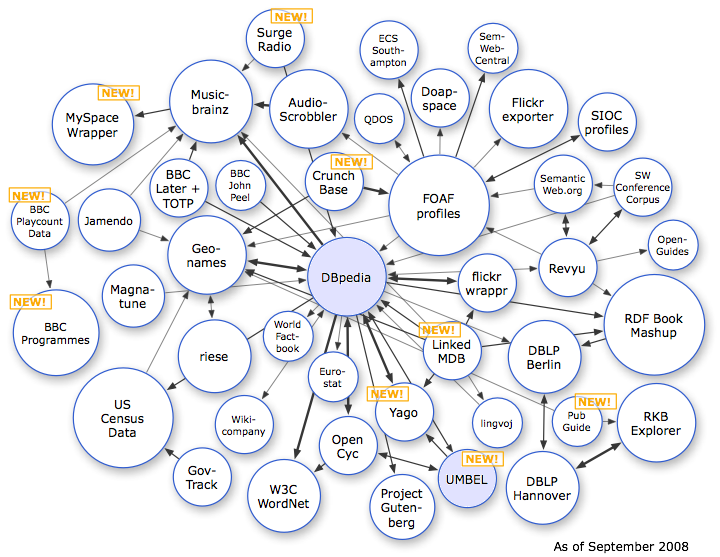
Class-level Mappings Now Generalize Semantic Web Connectivity
We are pleased to present a complementary view to the now-famous linking open data (LOD) cloud diagram (shown to the left; click on it for a full-sized view) [1]. This new diagram (shown below) — what we call the LOD constellation to distinguish it from its notable LOD cloud sibling — presents the current class-level structure within LOD datasets.
This new LOD constellation complements the instance-level view in the LOD cloud that has been the dominant perspective to date. The LOD cloud centrally hubs around DBpedia, the linked data structured representation of Wikipedia. The connections shown in the cloud diagram mostly reflect owl:sameAs relations, which means that the same individual things or instances are referenced and then linked between the datasets. Across all datasets, linking open data (LOD) now comprises some two billion RDF triples, which are interlinked by around 3 million RDF links [2]. This instance-level view of the LOD cloud shown to the left was updated a bit over a week ago [1].
The objective of the Linking Open Data community is to extend the Web with a data commons by publishing various open datasets as RDF on the Web and by setting RDF links between data items from different data sources. All of the sources on these LOD diagrams are open data [3].
So, Tell me Again Why Classes Are Important?
In prior postings, Fred Giasson and I have explained the phenomenon of ‘exploding the domain‘. Exploding the domain means to make class-to-class mappings between a reference ontology and external ontologies, which allows properties, domains and ranges of applicability to be inherited under appropriate circumstances [4]. Exploding the domain expands inferencing power to this newly mapped information. Importantly, too, exploding the domain also means that instances or individuals that are members of these mapped classes also inherit or assume the structural relations (schema, if you will) of their mapped sources as well.
Trying to think through the statements above, however, is guaranteed to make your head hurt. When just reading the words, these sound like fairly esoteric or abstract ideas.
So, to draw out the distinctions, let’s discuss linked data that is based on instance (individual) mappings versus those mapped on the basis of classes. Like all things, there are exceptions and edge cases, but let us simply premise our example using basic set theory. Our individual instances are truly discrete individuals, in this case some famous dogs, and our classes are the conceptual sets by which these individuals might be characterized.
To make our example simple, we will use two datasets (A and B) about dogs and their possible relations, each characterized by their structure (classes) or their individuals (instances):
|
Dataset A (organisms) |
Dataset B (pets) |
Classes (structure) |
mammal
canid
wolf
dog |
pet
dog
breed (list) |
Instances (individuals) and class assignments |
Rin Tin Tin (dog) |
Rin Tin Tin (German shepherd)
Lassie (collie)
Clifford (Visla)
Old Yeller (mutt) |
When datasets are linked based on instance mappings alone, as is generally the case with current practice using sameAs, and there are no class mappings, we can say that Rin Tin Tin is both a dog pet and a mammal. However, we can not say that Lassie, for example, is a mammal, because there is no record for Lassie in Dataset A.
So, we thus see our first lesson: to draw an inference about instances using sameAs in the absence of class mappings requires each record (instance) to exist in an external dataset in order to make that assertion. Instances can inherit the properties and structure of the datasets in which they specifically occur, but only there. Thus, what can be said about a given individual (linked via owl:sameAs) is at most the intersection of what is contained in only the datasets in which that individual appears and is mapped. Assertions are thus totally specific and can not be made without the presence of a matching instance record. We can call this scenario the intersection model: only where there is an intersection of matching instance records can the structure of their source datasets be inferred.
However, when mappings can be made at the class level, then inferences can be drawn about all of the members of those sets. By asserting equivalentClass for dog between Datasets A and B, we can now infer that Lassie, Clifford and Old Yeller are canids and mammals as well as Rin Tin Tin, even though their instance records are not part of Dataset A. To complete the closure we can also now infer that Rin Tin Tin (Dataset A) is a pet and a German shepherd from Dataset B. We can call this scenario the union model. The mappings have become generalized and our inferencing power now extends to all instances of mapped classes whether there are records or not for them in other datasets.
This power of generalizability, plus the inheritance of structure, properties and domain and range attributes, is why class mappings are truly essential for the semantic Web. Exploding the domain is real and powerful. Thus, to truly understand the power of linked data, it is necessary to view its entirety from a class perspective [5].
Thus, to summarize our answer to the rhetorical question, class mappings are important because they can:
- Generalize the understanding of individual instances
- Expand the description of things in the world by inheriting and reusing other class properties, domains and ranges, and
- Place and contextualize things by inheriting class structure and hierarchical relationships.
The LOD Constellation
So, here is the new LOD constellation of class-level linkages. The definition of class-level linkages is based on one of four possible predicates (rdfs:subClassOf, owl:equivalentClass, umbel:superClassOf or umbel:isAligned). Because of the newness of UMBEL as a vocabulary, only a few of the sources linked to UMBEL have the umbel:superClassOf relationship and one (bibo) has isAligned.
Note that some of the sources are combined vocabularies (ontologies) and instance representations (e.g., UMBEL, GeoNames), others are strict ontologies (e.g., event, bibo), and still others are ontologies used to characterize distributed instances (e.g., foaf, sioc, doap). Other distinctions might be applied as well:
[click for full size]
The current 21 LOD datasets and ontologies that contribute to these class-level mappings are (with each introduced by its namespace designation):
- bibo — Bibilographic ontology
- cc — Creative Commons ontology
- damltime — Time Zone ontology
- doap — Description of a Project ontology
- event — Event ontology
- foaf — Friend-of-a-Friend ontology
- frbr — Functional Requirements for Bibliographic Records
- geo — Geo wgs84 ontology
- geonames — GeoNames ontology
- mo — Music Ontology
- opencyc — OpenCyc knowledge base
- owl — Web Ontology Language
- pim_contact — PIM (personal information management) Contacts ontology
- po — Programmes Ontology (BBC)
- rss — Really Simple Syndicate (1.0) ontology
- sioc — Socially Interlinked Online Communities ontology
- sioc_types — SIOC extension
- skos — Simple Knowledge Organization System
- umbel — Upper Mapping and Binding Exchange Layer ontology
- wordnet — WordNet lexical ontology
- yandex_foaf — FOAF (Friend-of-a-Friend) Yandex extension ontology
The diagram was programmatically generated using Cytoscape (see below) [6], with some minor adjustments in bubble position to improve layout separation. The bubble sizes are related to number of linked structures (ontologies) to which the node has class linkages. The arrow thicknesses are related to number of linking predicates between the nodes. Two-way arrows are shown as darker and indicate equivalentClass or matching superClassOf and subClassOf; single arrows represent subClassOf relationships only.
Note we are not presenting any rdf:type relations because those are not structural, and rather deal with the assignment of instances to classes [7]. More background is provided in the discussion of the construction methodology [6].
At this time, we have not calculated how many individuals or instances might be directly included in these class-level mappings. The data and files used in constructing this diagram are available for download without restriction [8].
Finally, we have expended significant effort to discover class-level mappings for which we may not be directly aware (see next). Please bring any missing, erroneous or added linkages to our attention. We will be pleased to incorporate those updates into future releases of the diagram.
How the LOD Constellation Was Constructed
Our diligence has not been exhaustive since not all LOD datasets are indexed locally and others do not have SPARQL endpoints. The general method was to query the datasets to check which ontologies used external classes to instantiate their individuals using the rdf:type predicate. The externally referenced ontology was then checked to determine its own external class mappings.
Here is the basic SPARQL query to discover the the rdf:type predicate for non-Virtuoso resources:
And here is the SPARQL query for Virtuoso-hosted datasets (note Virtuoso supports the distinct non-standard extension to SPARQL which is a more efficient way to get listings):
We then created a simple script to go to all of the ontology namespaces so listed in these external mappinigs. If there was an external class mapping in the source with one of the four possible predicates of rdfs:subClassOf, owl:equivalentClass, umbel:superClassOf or umbel:isAligned, we noted the source and predicate and wrote it to a simple CSV (comma delimited) file. This formed the input file to the Cytoscape program that created the network graph [6].
There are possibly oversights and omissions in this first-release diagram since not all bubbles in the LOD cloud were exhaustively inspected. Please notify us with updates or new class linkages. Alternatively, you can also download and modify the diagram yourself [8].
Conspicuous by Their Absence
We gave particular diligence to a few of the more dominant sources in the LOD instance cloud that showed no class mappings. These include DBpedia and YAGO. While these have numerous and useful rdf:type and owl:sameAs relationships, and all have rich internal class structures, none apparently map at the class level to external sources.
However, because of the unique overlap of instances (named entities) in UMBEL, which does have extensive external class mappings, and which have been mapped to DBpedia instances, it is possible to infer some of these external class linkages.
For example, go to the DBpedia SPARQL endpoint:
http://dbpedia.org/sparql/
And try out some sample queries by pasting the following into the Query text box and running the query:
This example query applies the external class structure of UMBEL to individual person instances in DBpedia because of the prior specification of some mapping rules used for inferencing [9]. The result set is limited to 1000 results.
Alternatively, since UMBEL has also been mapped to the external FOAF ontology, we can also now invoke the FOAF class structure directly to produce the exact same result set (since umbel:Person owl:equivalentClass foaf:Person). We do this by applying the same inferencing rules in a different way:
UMBEL can thus effectively act as a class structure bridge to the DBpedia instances.
Since DBpedia is an instance hub, this bridging effect is quite effective between UMBEL and other DBpedia instances in the LOD cloud. However, because there is not the same degree of overlap of instances with, say, GeoNames, this technique would be less effective there.
Explicit class-level mappings between datasets will always be more powerful than instance-level ones with class mediators. And, in all cases, both of those techniques that explicitly invoke classes are more powerful than instance-level links alone.
The Linked Data Infrastructure is Now Complete
Though all of the available linkages have not yet been made in the LOD datasets, we can now see that all essential pieces of the linkage infrastructure are in place and ready to be exploited. Of course, new datasets can take advantage of this infrastructure as well.
UMBEL is one of the essential pieces that provides the bridging “glue” to these two perspectives or “worlds” of the instances in the LOD cloud and the classes in the LOD constellation. This “glue” becomes possible because of UMBEL’s unique combination of three components or roles:
- UMBEL provides a rich set of 20,000 subject concept classes and their relations (a reference structure “backbone”) that facilitates class-level mappings with virtually any external ontology with the benefits as described above
- UMBEL contains a named entity dictionary from Wikipedia also mapped to these classes, which therefore strongly intersects with DBpedia and YAGO, and therefore helps provide the individual instances <–> classes bridging “glue”, and
- UMBEL is also a vocabulary that enhances the lightweight SKOS vocabulary to explicitly facilitate linkages to external ontologies at the subject concept layer.
In fact, it is the latter vocabulary sense, in combination with the reference subject concepts, that enables us to draw the LOD class constellation.
So, we can now see a merger of the LOD cloud and the LOD constellation to produce all of the needed parts to the LOD infrastructure for going forward:
- A hub of instances (DBpedia)
- A hub of subject-oriented (“is about”) reference classes (Cyc-UMBEL), and
- A vocabulary for glueing it all together (SKOS-UMBEL).
This infrastructure is ready today and available to be exploited for those who can grasp its game-changing significance.
And, from UMBEL’s standpoint, we can also point to the direct tie-ins to the Cyc knowledge base and structure for conceptual and relationship coherence testing. This infrastructure is an important enabler to extend these powerful frameworks to new domains and for new purposes. But that is a story for another day. 😉
[2] According to [1], this estimate was last updated one year ago in October 2007. The numbers today are surely much larger, since the number of datasets has also nearly doubled in the interim.
[3]
Open data has many definitions, but a common one with a
badge is often seen. However, the best practices of linked data can also be applied to proprietary or intranet information as well; see
this FAQ.
[5] Of course, instance-level mappings with
sameAs have value as well, as the usefulness of linked data to date demonstrates. The point is not that class-level mappings are the “right” way to construct linked data. Both instance mappings and class mappings complement one another, in combination bringing both
specificity and
generalizability. The polemic here is merely to redress today’s common oversight of the class-level perspective.
[6] See the text for how the listing of class relationships was first assembled. After removal of duplicates, a simple comma delimited file was produced, class_level_lod_constellation.csv, with four columns. The first three columns show the subject-predicate-object linking the datasets by the class-level predicate. The fourth column presents the count of the number of types of class-level predicates used between the dataset pairs; the maximum value is 4
.
This CVS file is the import basis to
Cytoscape. After import, the spring algorithm was applied to set the initial distribution of nodes (bubbles) in the diagram. Styles were developed to approximate the style in the LOD
cloud diagram, and each of the class-linkage predicates was assigned different views and arrows. (The scaling of arrow width allowed the chart to be cleaned up with repeat linkages removed and simplified to a single arrow, with the strongest link type used as the remaining assignment. For example,
equivalentClass is favored over
subClassOf is favored over
superClassOf.)
In addition, each node was scaled according to the number of external dataset connections, with the assignments as shown in the file. Prior to finalizing, the node bubbles were adjusted for clear spacing and presentation. The resulting output is provided as the Cytoscape file, lod_class_constellation.cys. For the published diagram, the diagram was also exported as an SVG file.
This SVG file was lastly imported into
Inkscape for final clean up. The method for constructing the final diagram, including discussion about how the shading effect was added, is available upon request.
[7]
rdf:type is a predicate for assigning an instance to a class, which is often an external class. It is an important source of linkages between datasets at the instance level and provides a structural understanding of the instance within its dataset. While this adds structural richness for instances,
rdf:type is by definition not class-level and provides no generalizability.