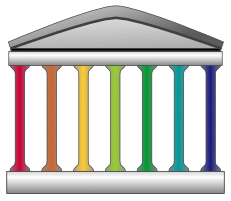
Guideposts for How to Make the Transition
The beginning of a new year and a new decade is a perfect opportunity to take stock of how the world is changing and how we can change with it. Over the past year I have been writing on many foundational topics relevant to the use of semantic technologies in enterprises.
In this post I bring those threads together to present a unified view of these foundations — some seven pillars — to the open semantic enterprise.
By open semantic enterprise we mean an organization that uses the languages and standards of the semantic Web, including RDF, RDFS, OWL, SPARQL and others to integrate existing information assets, using the best practices of linked data and the open world assumption, and targeting knowledge management applications. It does so using some or all of the seven foundational pieces (“pillars”) noted herein.
The foundational approaches to the open semantic enterprise do not necessarily mean open data nor open source (though they are suitable for these purposes with many open source tools available [3]). The techniques can equivalently be applied to internal, closed, proprietary data and structures. The techniques can themselves be used as a basis for bringing external information into the enterprise. ‘Open’ is in reference to the critical use of the open world assumption.
These practices do not require replacing current systems and assets; they can be applied equally to public or proprietary information; and they can be tested and deployed incrementally at low risk and cost. The very foundations of the practice encourage a learn-as-you-go approach and active and agile adaptation. While embracing the open semantic enterprise can lead to quite disruptive benefits and changes, it can be accomplished as such with minimal disruption in itself. This is its most compelling aspect.
Like any change in practice or learning, embracing the open semantic enterprise is fundamentally a people process. This is the pivotal piece to the puzzle, but also the one that does not lend itself to ready formula about pillars or best practices. Leadership and vision is necessary to begin the process. People are the fuel for impelling it. So, we’ll take this fuel as a given below, and concentrate instead on the mechanics and techniques by which this vision can be achieved. In this sense, then, there are really eight pillars to the open semantic enterprise, with people residing at the apex.
This article is synthetic, with links to (largely) my preparatory blog postings and topics that preceded it. Assuming you are interested in becoming one of those leaders who wants to bring the benefits of an open semantic enterprise to your organization, I encourage you to follow the reference links for more background and detail.
A Review of the Benefits
OK, so what’s the big deal about an open semantic enterprise and why should my organization care?
We should first be clear that the natural scope of the open semantic enterprise is in knowledge management and representation [1]. Suitable applications include data federation, data warehousing, search, enterprise information integration, business intelligence, competitive intelligence, knowledge representation, and so forth [2]. In the knowledge domain, the benefits for embracing the open semantic enterprise can be summarized as greater insight with lower risk, lower cost, faster deployment, and more agile responsiveness.
The intersection of knowledge domain, semantic technologies and the approaches herein means it is possible to start small in testing the transition to a semantic enterprise. These efforts can be done incrementally and with a focus on early, high-value applications and domains.
There is absolutely no need to abandon past practices. There is much that can be done to leverage existing assets. Indeed, those prior investments are often the requisite starting basis to inform semantic initiatives.
Embracing the pillars of the open semantic enterprise brings these knowledge management benefits:
- Domains can be analyzed and inspected incrementally
- Schema can be incomplete and developed and refined incrementally
- The data and the structures within these frameworks can be used and expressed in a piecemeal or incomplete manner
- Data with partial characterizations can be combined with other data having complete characterizations
- Systems built with these frameworks are flexible and robust; as new information or structure is gained, it can be incorporated without negating the information already resident, and
- Both open and closed world subsystems can be bridged.
Moreover, by building on successful Web architectures, we can also put in place loosely coupled, distributed systems that can grow and interoperate in a decentralized manner. These also happen to be perfect architectures for flexible collaboration systems and networks.
These benefits arise both from individual pillars in the open semantic enterprise foundation, as well as in the interactions between them. Let’s now re-introduce these seven pillars.
Pillar #1: The RDF Data Model
As I stated on the occasion of the 10th birthday of the Resource Description Framework data model, I belief RDF is the single most important foundation to the open semantic enterprise [4]. RDF can be applied equally to all structured, semi-structured and unstructured content. By defining new types and predicates, it is possible to create more expressive vocabularies within RDF. This expressiveness enables RDF to define controlled vocabularies with exact semantics. These features make RDF a powerful data model and language for data federation and interoperability across disparate datasets.
Via various processors or extractors, RDF can capture and convey the metadata or information in unstructured (say, text), semi-structured (say, HTML documents) or structured sources (say, standard databases). This makes RDF almost a “universal solvent” for representing data structure.
Because of this universality, there are now more than 150 off-the-shelf ‘RDFizers’ for converting various non-RDF notations (data formats and serializations) to RDF [5]. Because of its diversity of serializations and simple data model, it is also easy to create new converters. Once in a common RDF representation, it is easy to incorporate new datasets or new attributes. It is also easy to aggregate disparate data sources as if they came from a single source. This enables meaningful compositions of data from different applications regardless of format or serialization.
What this practically means is that the integration layer can be based on RDF, but that all source data and schema can still reside in their native forms [6]. If it is easier or more convenient to author, transfer or represent data in non-RDF forms, great [7]. RDF is only necessary at the point of federation, and not all knowledge workers need be versed in the framework.
Pillar #2: Linked Data Techniques
Linked data is a set of best practices for publishing and deploying instance and class data using the RDF data model. Two of the best practices are to name the data objects using uniform resource identifiers (URIs), and to expose the data for access via the HTTP protocol. Both of these practices enable the Web to become a distributed database, which also means that Web architectures can also be readily employed (see Pillar #5 below).
Linked data is applicable to public or enterprise data, open or proprietary. It is really straightforward to employ. Structured Dynamics has published a useful FAQ on linked data.
Additional linked data best practices relate to how to characterize and classify data, especially in the use of predicates with the proper semantics for establishing the degree of relatedness for linked data items from disparate sources.
Linked data has been a frequent topic of this blog, including how adding linkages creates value for existing data, with a four-part series about a year ago on linked data best practices [8]. As advocated by Structured Dynamics, our linked data best practices are geared to data interconnections, interrelationships and context that is equally useful to both humans and machine agents.
Pillar #3: Adaptive Ontologies
Ontologies are the guiding structures for how information is interrelated and made coherent using RDF and its related schema and ontology vocabularies, RDFS and OWL [10]. Thousands of off-the-shelf ontologies exist — a minority of which are suitable for re-use — and new ones appropriate to any domain or scope at hand can be readily constructed.
In standard form, semantic Web ontologies may range from the small and simple to the large and complex, and may perform the roles of defining relationships among concepts, integrating instance data, orienting to other knowledge and domains, or mapping to other schema [11]. These are explicit uses in the way that we construct ontologies; we also believe it is important to keep concept definitions and relationships expressed separately from instance data and their attributes [9].
But, in addition to these standard roles, we also look to ontologies to stand on their own as guiding structures for ontology-driven applications (see next pillar). With a relatively few minor and new best practices, ontologies can take on the double role of informing user interfaces in addition to standard information integration.
In this vein we term our structures adaptive ontologies [11,12,13]. Some of the user interface considerations that can be driven by adaptive ontologies include: attribute labels and tooltips; navigation and browsing structures and trees; menu structures; auto-completion of entered data; contextual dropdown list choices; spell checkers; online help systems; etc. Put another way, what makes an ontology adaptive is to supplement the standard machine-readable purpose of ontologies to add human-readable labels, synonyms, definitions and the like.
A neat trick occurs with this slight expansion of roles. The knowledge management effort can now shift to the actual description, nature and relationships of the information environment. In other words, ontologies themselves become the focus of effort and development. The KM problem no longer needs to be abstracted to the IT department or third-party software. The actual concepts, terminology and relations that comprise coherent ontologies now become the explicit focus of KM activities.
Any existing structure (or multiples thereof) can become a starting basis for these ontologies and their vocabularies, from spreadsheets to naïve data structures and lists and taxonomies. So, while producing an operating ontology that meets the best practice thresholds noted herein has certain requirements, kicking off or contributing to this process poses few technical or technology demands.
The skills needed to create these adaptive ontologies are logic, coherent thinking and domain knowledge. That is, any subject matter expert or knowledge worker likely has the necessary skills to contribute to useful ontology development and refinement. With adaptive ontologies powering ontology-driven apps (see next), we thus see a shift in roles and responsibilities away from IT to the knowledge workers themselves. This shift acts to democratize the knowledge management function and flatten the organization.
Pillar #4: Ontology-driven Applications
The complement to adaptive ontologies are ontology-driven applications. By definition, ontology-driven apps are modular, generic software applications designed to operate in accordance with the specifications contained in an adaptive ontology. The relationships and structure of the information driving these applications are based on the standard functions and roles of ontologies, as supplemented by the human and user interface roles noted above [11,12,13].
Ontology-driven apps fulfill specific generic tasks. Examples of current ontology-driven apps include imports and exports in various formats, dataset creation and management, data record creation and management, reporting, browsing, searching, data visualization, user access rights and permissions, and similar. These applications provide their specific functionality in response to the specifications in the ontologies fed to them.
The applications are designed more similarly to widgets or API-based frameworks than to the dedicated software of the past, though the dedicated functionality (e.g., graphing, reporting, etc.) is obviously quite similar. The major change in these ontology-driven apps is to accommodate a relatively common abstraction layer that responds to the structure and conventions of the guiding ontologies. The major advantage is that single generic applications can supply shared functionality based on any properly constructed adaptive ontology.
This design thus limits software brittleness and maximizes software re-use. Moreover, as noted above, it shifts the locus of effort from software development and maintenance to the creation and modification of knowledge structures. The KM emphasis can shift from programming and software to logic and terminology [12].
Pillar #5: A Web-oriented Architecture
A Web-oriented architecture (WOA) is a subset of the service-oriented architectural (SOA) style, wherein discrete functions are packaged into modular and shareable elements (”services”) that are made available in a distributed and loosely coupled manner. WOA uses the representational state transfer (REST) style. REST provides principles for how resources are defined and used and addressed with simple interfaces without additional messaging layers such as SOAP or RPC. The principles are couched within the framework of a generalized architectural style and are not limited to the Web, though they are a foundation to it [14].
REST and WOA stand in contrast to earlier Web service styles that are often known by the WS-* acronym (such as WSDL, etc.). WOA has proven itself to be highly scalable and robust for decentralized users since all messages and interactions are self-contained.
Enterprises have much to learn from the Web’s success. WOA has a simple design with REST and idempotent operations, simple messaging, distributed and modular services, and simple interfaces. It has a natural synergy with linked data via the use of URI identifiers and the HTTP transport protocol. As we see with the explosion of searchable dynamic databases exposed via the Web, so too can we envision the same architecture and design providing a distributed framework for data federation. Our daily experience with browser access of the Web shows how incredibly diverse and distributed systems can meaningfully interoperate [15].
This same architecture has worked beautifully in linking documents; it is now pointing the way to linking data; and we are seeing but the first phases of linking people and groups together via meaningful collaboration. While generally based on only the most rudimentary basis of connections, today’s social networking platforms are changing the nature of contacts and interaction.
The foundations herein provide a basis for marrying data and documents in a design geared from the ground up for collaboration. These capabilities are proven and deployable today. The only unclear aspects will be the scale and nature of the benefits [16].
Pillar #6: An Incremental, Layered Approach
To this point, you’ll note that we have been speaking in what are essentially “layers”. We began with existing assets, both internal and external, in many diverse formats. These are then converted or transformed into RDF-capable forms. These various sources are then exposed via a WOA Web services layer for distributed and loosely-coupled access. Then, we integrate and federate this information via adaptive ontologies, which then can be searched, inspected and managed via ontology-driven apps. We have presented this layered architecture before [13], and have also expressed this design in relation to current Structured Dynamics’ products [17].
A slight update of this layered view is presented below, made even more general for the purposes of this foundational discussion:
(click to expand)
Semantic technology does not change or alter the fact that most activities of the enterprise are transactional, communicative or documentary in nature. Structured, relational data systems for transactions or records are proven, performant and understood. On its very face, it should be clear that the meaning of these activities — their semantics, if you will — is by nature an augmentation or added layer to how to conduct the activities themselves.
This simple truth affirms that semantic technologies are not a starting basis, then, for these activities, but a way of expressing and interoperating their outcomes. Sure, some semantic understanding and common vocabularies at the front end can help bring consistency and a common language to an enterprise’s activities. This is good practice, and the more that can be done within reason while not stifling innovation, all the better. But we all know that the budget department and function has its own way of doing things separate from sales or R&D. And that is perfectly OK and natural.
Clearly, then, an obvious benefit to the semantic enterprise is to federate across existing data silos. This should be an objective of the first semantic “layer”, and to do so in a way that leverages existing information already in hand. This approach is inherently incremental; if done right, it is also low cost and low risk.
Pillar #7: The Open World Mindset
As these pillars took shape in our thinking and arguments over the past year, an illusive piece seemed always to be missing. It was like having one of those meaningful dreams, and then waking up in the morning wracking your memory trying to recall that essential, missing insight.
As I most recently wrote [1], that missing piece for this story is the open world assumption (OWA). I argue that this somewhat obscure concept holds within it the key as to why there have been decades of too-frequent failures in the enterprise in business intelligence, data warehousing, data integration and federation, and knowledge management.
Enterprises have been captive to the mindset of traditional relational data management and its (most often unstated) closed world assumption (CWA). Given the success of relational systems for transaction and operational systems — applications for which they are still clearly superior — it is understandable and not surprising that this same mindset has seemed logical for knowledge management problems as well. But knowledge and KM are by their nature incomplete, changing and uncertain. A closed-world mindset carries with it certainty and logic implications not supportable by real circumstances.
This is not an esoteric point, but a fundamental one. How one thinks about the world and evaluates it is pivotal to what can be learned and how and with what information. Transactions require completeness and performance; insight requires drawing connections in the face of incompleteness or unknowns.
The absolute applicability of the semantic Web stack to an open-world circumstance is the elephant in the room [1]. By itself, the open world mindset provides no assurance of gaining insight or wisdom. But, absent it, we place thresholds on information and understanding that may neither be affordable nor achievable with traditional, closed-world approaches.
And, by either serendipity or some cosmic beauty, the open world mindset also enables incremental development, testing and refinement. Even if my basic argument of the open world advantage for knowledge management purposes is wrong, we can test that premise at low cost and risk. So, within available budget, pick a doable proof-of-concept, and decide for yourself.
The Foundations for the Open Semantic Enterprise
The seven pillars above are not magic bullets and each is likely not absolutely essential. But, based on today’s understandings and with still-emerging use cases being developed, we can see our open semantic enterprise as resulting from the interplay of these seven factors:
Thirty years of disappointing knowledge management projects and much wasted money and effort compel that better ways must be found. On the other hand, until recently, too much of the semantic Web discussion has been either revolutionary (“change everything!!”) or argued from pie-in-the-sky bases. Something needs to give.
Our work over the past few years — but especially as focused in the last 12 months — tells us that meaningful semantic Web initiatives can be mounted in the enterprise with potentially huge benefits, all at manageable risks and costs. These seven pillars point to way to how this might happen. What is now required is that eighth pillar — you.
[2] In most instances, semantic technologies are poorly suited to transactional or operational applications. Also, there are instances in modeling specific closed-world domains where ontologies can be quite useful, such as in aerospace, petrochemicals, engineering, etc., where the scope of the domain can be precisely bounded and defined. Such efforts tend to be high cost with lengthy lead times. There are vendors who support efforts in these areas, though my company,
Structured Dynamics, does not. Our focus and the more generally suitable case for semantic technologies we believe is in knowledge representation and management.
[3] The standard
Sweet Tools listing on my
AI3:::Adaptive Information blog contains more than 800 semantic Web and -related tools, most of which are open source, which can be inspected via filtered and faceted search.
[5] For example, see this listing of more than 150 specific
format options available as open source. These converters can also work directly with major application APIs.
[7] For example, for dataset authoring, Structured Dynamics has developed
irON, an instance record and object notation that can be serialized as JSON (called
irJSON), XML (called
irXML) or comma-separated values (or CSV comma-delimited files, called
commON). The purpose of these notations is to provide easier authoring environments and scripting support to RDF-ready datasets. The advantage is to shield users from the nuances of RDF. The design of
commON is especially geared to using spreadsheets as authoring environments for instance record tables or simple outline structures. See further the
irON specification.
[9] Our best practices approach makes explicit splits between the “ABox” (for instance data) and “TBox” (for ontology schema) in accordance with our working definition for description logics, a fundamental underpinning for how we use RDF:
“Description logics and their semantics traditionally split concepts and their relationships from the different treatment of instances and their attributes and roles, expressed as fact assertions. The concept split is known as the TBox (for terminological knowledge, the basis for T in TBox) and represents the schema or taxonomy of the domain at hand. The TBox is the structural and intensional component of conceptual relationships. The second split of instances is known as the ABox (for assertions, the basis for A in ABox) and describes the attributes of instances (and individuals), the roles between instances, and other assertions about instances regarding their class membership with the TBox concepts.”