A Peek at Forthcoming Topics in the Cooking with Python and KBpedia Series
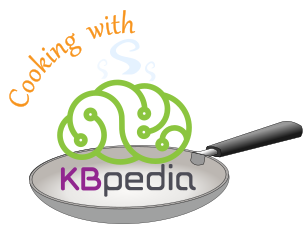
Throughout this Cooking with Python and KBpedia series I want to cover some major topics about how to use, maintain, build, and extend knowledge graphs, using our KBpedia knowledge system as the model. KBpedia is a good stalking horse for these exercises — really, more like recipes — because it has broad domain coverage and a modular design useful for modification or extension for your own domain purposes. At appropriate times throughout these exercises you may want to fork your own version of KBpedia and the accompanying code to whack at for your own needs.
Today, as I begin my first articles, I am anticipating scores of individual installments in our CWPK series. Some 55 of these installments and the associated code have already been written. Though the later portion of the entire series gets more complicated, I am hoping that the four months or so it will take to publish the anticipated 75 installments at the rate of one per business day will give me sufficient time to complete the series in time. We shall see.
My intent is not to provide a universal guide, since I will be documenting steps using a specific knowledge graph, a specific environment (Windows 10 and some AWS Ubuntu), and a specific domain (knowledge management and representation). As the series name defines, we will only be working with the Python language. We’ve made these choices because of familiarity, and our desire to produce a code base at the series’ conclusion that better enables users to modify and extend KBpedia from scratch. We also are focusing on the exploring newbie more than the operating enterprise. I do not touch on issues of security, code optimization, or scalability. My emphasis is more on simplicity and learning, not performance or efficiency. Those with different interests may want to skip some installments and consult the suggested resources. Still, truly to my knowledge, there is nothing like this series out there: a multiple installment, interactive and didactive environment for learning ‘soup-to-nuts‘ practical things about semantic technologies and knowledge graphs.
The first part of the installments deals with the design intent of the series, architecture, and selection and installation of tools and applications. Once we have the baseline system loaded and working, we explore basic use and maintenance tasks for a knowledge graph. We show how those are done with a common ontology development environment, the wonderful Protégé open-source IDE, as well as programmatically through Python. At various times we will interact with the knowledge base using Python programmatically or via the command line, electronic notebooks, or Web page templates. There are times when Protégé is absolutely the first tool of choice. But to extract the most value from a knowledge graph we also need to drive it programmatically, sometimes analyze it as a graph, do natural language tasks, or use it to stage training sets or corpora for supervised or unsupervised maching learning. Further, to best utilize knowledge graphs, we need to embed them in our daily workflows, which means interacting with them in a distributed, multiple-application way, as opposed to a standalone IDE. This is why we must learn tools that go beyond navigation and inspection. The scripts we will learn from these basic use and maintenance tasks will help us get famiiar with our new Python surroundings and to set a foundation for next topics.
In our next part, we begin working with Python in earnest. Of particular importance is finding a library or set of APIs to work directly with OWL, the language of the KBpedia knowledge graph. There are many modifications and uses of KBpedia that existing Python tools can aid. Having the proper interface or API to talk directly to the object types within the knowledge graph is essential. There are multiple options for how to approach this question, and no single, ‘true’ answer. Once we have selected and installed this library, we then need to sketch out the exact ways we intend to access, use and modify KBpedia. These actions then set our development agenda for finding and scripting Python tools into our workflow.
There are off-the-shelf Python tools for querying the knowledge graph (SPARQL), adding rules to the graph (SWRL), and visualizing the outputs. Because we are also using Python to manipulate KBpedia, we also need to understand how to write outputs to file, necessary as inputs to other third-party tools and advanced applications. Since this is the first concentrated section involved in finding and modifying existing Python code, we’ll also use a couple of installments to assemble and document useful Python coding fragments and tips.
Armed with this background, and having gotten our feet wet a bit with Python, we are now positioned to begin writing our own Python code to achieve our desired functionality. We begin this process by focusing on some standard knowledge graph modification tasks: adding or changing nodes, properties or labels (annotations) within our knowledge graph. Of course, these capabilities are available directly from Protégé. However, we want to develop our own codes for this process in line with how we build and test these knowledge graphs in the first place. These skills set the foundation for how we can filter and make subset selections as training sets for machine learning. One key installment from this part of the CWPK series involves how we set up comma-separated values (CSV) files in UTF-8 format, the standard we have adopted for staging for use by third-party tools and in KBpedia’s build process. We also discuss the disjoint property in OWL and its relation to the modular typology design used by KBpedia. Via our earlier Python-OWL mappings we will see how we can use the Python language itself to manipulate these OWL constructs.
The installments in this initial part of the series are about setting up and learning about our tools and environment so that we can begin doing new, real work against our knowledge graphs. The first real work to which we will apply these tools is the extraction-and-build routines by which we can produce a knowledge graph from scratch. One of the unusual aspects of KBpedia is that the knowledge graph, as large and as comprehensive as it may be, is built entirely from a slate of flat (CSV, in N3 format) input files. KBpedia, in its present version 2.50, has about five standard input files, plus five optional files for various fixes or overrides, and about 30 external mapping files (which vary, obviously, by the number of external sources we integrate). These files can be easily and rapidly edited and treated in bulk, which are then used as the inputs to the build process. The build process also integrates a number of syntax and logical checks to make sure the finally completed knowledge graph is consistent and satisfiable, with standardized syntax. As errors are surfaced, modifications get made, until the build finally passes its logic tests. Multiple build iterations are necessary for any final public release. One of the reasons we wanted a more direct Python approach in this series was to bootstrap the routines and code necessary to enable this semi-automated build process. The build portion, in particular, has been part of KBpedia’s special sauce from the beginning, but is a capability we have not yet documented nor shared with the world. Since its beginning in 2018, each release of KBpedia has been the result of multiple build cycles to produce a tested, vetted knowledge graph.
In exposing these standard methods, we also needed to add to them a complementary set of extraction routines. Getting familiar with extracting resources from a knowledge graph has many practical applications and is an easier way to start learning Python. This substantive portion of CWPK is new, and gives us the ability to break down a knowledge graph into a similar set of simple, constituent input files. We thus end up with a unique roundtripping environment that, while specific to KBpedia as expressed in this series, can be modified though the Python code that accompanies this series for potentially any knowledge graph. It has also resulted in a more generalized approach suitable for other knowledge graphs than our original internal design supported. We now have a re-factored, second-generation set of knowledge graph extract-and-build routines. Some modifications may be needed for other types of starting knowledge graphs, but we hope the steps documented can be readily adapted for those purposes based on how we have described and coded them. Because of these advances, we will witness a new version release of KBpedia along this path.
The last major portion of installments provides some recipes on how to use and leverage a knowledge graph. A portion of those installments involve creating machine learning training sets and corpora. We will tie into some leading Python applications and libraries to conduct a variety of supervised and unsupervised learning tasks including categorization, clustering, fine-grained entity recognition, and sentiment and relation extraction. We will touch upon the leading Python toolsets available, and gain recipes for general ways to work with these systems. We will generate and use graph and word embedding models to also support those tasks, plus new ones like summarization. We will undertake graph analytics and do random walks over the KBpedia graph to probe network concepts like community, influence, and centrality. We will tie into general natural language processing (NLP) toolkits and show the value for these uses that may be extracted from KBpedia. This rather lengthy part of our series includes how to set up an endpoint for our knowledge graph and how to tie into graphing and charting libraries for various visualizations.
Since I am not a professional programmer and certainly am no expert in Python, the codes that are produced and distributed in this series are intended as starting off points. Perhaps others may develop more polished and performant code for these purposes over time. I welcome such input and will do what we can can to bring awareness and distribution mechanisms to any such improvements. But, crude and simple as they may be, all of the Python tools we build during this series, plus the instructions in the why and how to do so as demonstrated through interactive Jupyter Notebooks, can help start you on the path to modify, delete, or extend what exists in KBpedia with your own domain graphs and data.
We devote the concluding installments in our CWPK series to how you may leverage these resources to tackle your own needs and domain. We also try to provide additional resources each step of the way to aid your own learning. In the aggregate, these installments cover an awful lot of ground. However, inch by inch, we will make reaching our end goal a cinch.