The New Paradigm of ‘Substantive Marketing’ for Innovative IT
This decade has clearly marked a sea change in the move of enterprise software from proprietary to open source, as I have recently discussed [1]. It is instructive that only a mere six years ago I was in heated fights with my then Board about open source; today, that seems so quaint and dated.
Also during this period many have noted how open source has changed the capital required to begin a new software startup [2]. Open source both provides the tooling and the components for cobbling together specialty apps and extensions. Six and seven and even eight figure startup costs common just a decade ago have now dropped to four or five figures. When we see the explosion of hundreds of thousands of smartphone apps we are seeing the glowing residue of these additional sea changes. Dropping startup costs by one to three orders of magnitude is truly democratizing innovation.
But something else has been going on that is changing the face of enterprise software (besides consolidation, another factor I also recently commented on). And that factor is “marketing”. Much less commentary is made about this change, but it, too, is greatly lowering costs and fundamentally changing market penetration strategies. That topic — and my personal experience with it — is the focus of this article.
The Obsolete Recent Past
Besides the few remaining big providers of enterprise software — like IBM, Oracle, HP, SAP — most vendors have totally remade their sales practices of just a few years ago. Large sales forces with big commissions and a year to two year sales cycles can no longer be justified when software license fees and the percentage maintenance annuities that flow from them are dropping rapidly. Today’s mantras are doing more with less and doing it faster, hardly consistent with the traditional enterprise software model. Sure, big enterprises, especially big government and big business, have large sunk costs in legacy systems that will continue to be milked by existing vendors. But the flow is constricting with longer-term trends clear to see. The old enterprise software model is obsolete.
Even if it were not dying, it is hard to square huge investments in sales and marketing when product development has become inexpensive and agile. The proliferation of three-letter marketing acronyms for branding “new” product areas and standard formulas for product hype of just a few years ago also feels old and dated. Cozy relationships with conventional trade press pundits and market analysts seem to be diminishing in importance, possibly because the authoritativeness of their influence is also diminishing. It is harder to justify market firm subscription costs when priority budget items are being cut and new information outlets have emerged.
In response to this, many developers have forsaken the enterprise market for the consumer one. Indeed enterprises themselves are looking more and more to the consumer sector and commodity apps for innovation and answers. But, still, problems unique to enterprises remain and how to effectively reach them in this brave new world is today’s marketing problem for enterprise software vendors.
Most entities today, when opining about these challenges, tend to emphasize the need for “laser focus” and “rifle-shot” targeting of prospects. The advice takes the form of: 1) emphasize well-defined verticals; 2) know your market well; and 3) target and go after your likely prospects. Prospect data mining and targeted ad analysis are the proferred elixirs.
But, there is little evidence such refined methods for prospect identification and targeting are really working. Like politicians doing focus groups and opinion polling to capture the desired “message” of their potential electorates, these are all still “push” models of marketing. Yet we are swamped with pushed messages and marketing everywhere we turn. The model is failing.
Besides message overload, there are two issues with laser targeting. First, despite all that we try to know about ready buyers (for enterprise software), we really don’t know if any particular individual is truly needful, in a position to buy, has the authority to buy, or is the right advocate to make the internal sell. Second, though the idea of “laser” carries with it the image of focus and not flailing, it is in fact expensive to identify the targets and send a focused message their way. Because of these issues, decay rates for laser prospects throughout conventional sales pipelines continue to rise.
A New Marketing Paradigm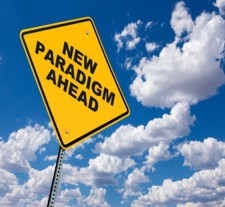
There has always been the phenomenon of the “fish jumping into the boat“; that is, the unanticipated inbound inquiry from a previously unknown prospect leading to a surprisingly swift sale. But we have seen this phenomenon increase markedly in recent years. Structured Dynamics‘ current customer base — including recurring customers — comes almost exclusively from this source. As we have noted this trend in comparison with more targeted outreach, we have spent much time trying to understand why it is occurring and how we can leverage what Peter Drucker called the “unexpected success” [3].
What we are seeing, I believe, is a shift from sales to marketing, and within marketing from direct or outbound marketing to a new paradigm of marketing. Others have likened this to inbound marketing [4] or content marketing [5] or permission marketing [6]. What we are seeing at Structured Dynamics bears many resemblances to parts of what is claimed for these other approaches, but not all. And, it is also true that what we are seeing may pertain mostly to innovative IT for emerging enterprise markets, and not a generalized paradigm suitable to other products or markets.
For lack of a better term, what we are seeing we can term “substantive marketing”. By this we mean offering valuable content and solutions-oriented systems for free and without restriction. This shares aspects with content marketing. Then, in keeping with the trend for buyers doing their own research and analysis to fulfill their own needs, similar to the premises of inbound or permission marketing, potential consumers can make their own judgments as to relevance and value of our offerings.
Sometimes, of course, some prospects find our approaches and solutions lacking. Sometimes, they may grab what we have offered for free and use them on their own without compensation to us. But where the match is right — and we need to be honest with both ourselves and the customer when it is not — we can better spend the customer’s limited time and resources to tailor our generic solutions to their specific needs. In doing so, we offer higher value (tailored services) while learning better about another spectrum of consumer need that can virtuously enhance our substantive offerings for the next prospect.
So, let’s decompose these components further to see what they can tell us about this new practice of substantive marketing and how to use it as an engine for moving forward.
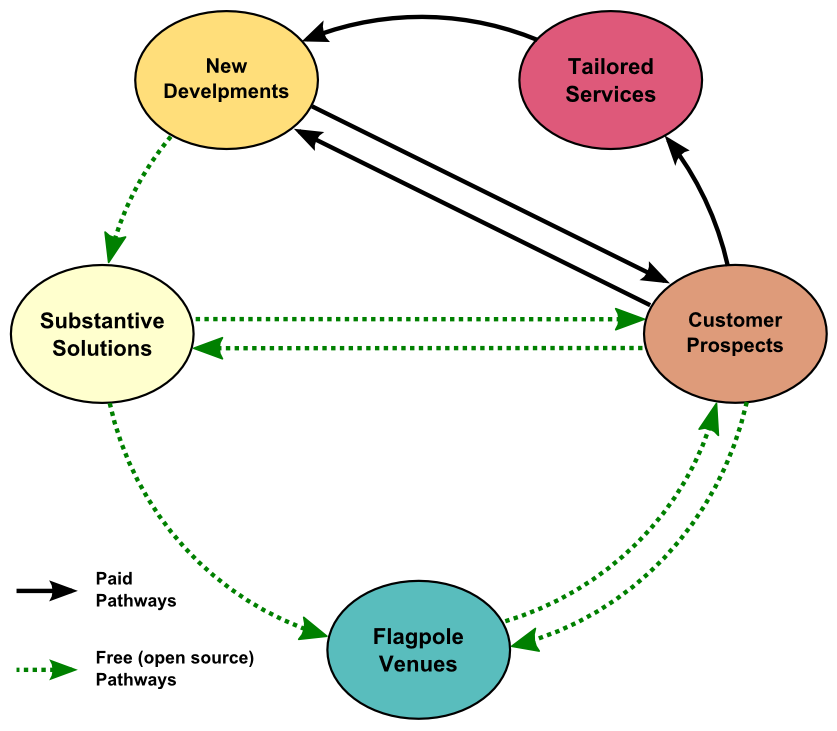
The Virtuous Cycle Begins with Substantive Solutions
The premise of substantive marketing is to offer square-deal value to the marketplace in the form of solutions-based content. Like content marketing that offers “the creation or sharing of content for the purpose of engaging current and potential consumer bases” [5], substantive marketing goes even further. The whole basis and premise of the approach is to provide substantive content, in one of more of these areas, preferably all:
- Knowledge — this substantive area includes papers, commentary, survey results or listings of tools and references useful to the target market
- Analysis — this content area includes unique analysis of market trends, data, technologies or reviews that pertain to the target market
- Code — this area relates to the provision of open source code and tools, preferably under licenses that allow users to use the software without restriction (two examples are the Apache 2 license and the MIT license)
- Documentation — a critical substantive area is the documentation in how to install, use, modify or customize these tools, including a prejudice to APIs and tutorial information
- Methodologies, workflows and best practices — it is important to also discuss how to properly operate and utilize these tools and information. Taking care to document lessons learned and best practices also helps the user community avoid common mistakes and to speed adoption and utility, and
- Demos — this area involves setting up (and sharing code and procedures for same) demos that show how the code and its methods actually work. Demos also become first use cases to aid the new user in learning and setting up the code bases.
Further, this substantive content is offered without strings, restrictions or customer fill-in forms. The content is not a come on or a teaser. We are not trying to gather leads or prospect names, because we have no intent to dun them with emails or follow-ups.
This substantive content is as complete as can be to enable new users to adopt the information and tools in their current state without further assistance. (In some cases, the information also educates the marketplace in order to prepare future customers for adoption.) Most importantly, this substantive content is offered for free, either open source (for code) or creative commons for documentation and other content. In return, it is fair to request — and we do — attribution when this material is used.
We have previously termed this complete panoply of substantive content a total open solution [7]. Some might find the provision of such robust information crazy: How can we give away the store of our proprietary knowledge and systems?
But we find this kind of thinking old school. In an open source world where so much information is now available online, with a bit of effort customers can find this information anyway. Rather, our mindset is that customers do not want to pay again for what has already been done, but are willing to pay for what can be done with that knowledge for their own specific problems. Offering the complete storehouse of our knowledge in fact signals our interest in only charging the customer for new answers, new value or new formulations. The customers we like to work with feel they are getting an honest, square deal.
Flagpole Venues Help Increase Awareness
Consider your substantive content to be your flag, a unique banner for conveying and packaging your specific brand. It is thus important to find appropriate flagpoles — in the virtual territories that your customers visit — for raising this content high for them to see. Since the role of these flagpoles is to create awareness in potential prospects — who you do not likely know individually or even by group in advance — it makes sense to raise your offerings up on many flagpoles and on the highest flagpoles. Visibility is the object of the approach.
This approach is distinctly not leafletting or cramming links or emails into as many spaces as possible. The idea of substantive marketing is to fly valuable content high enough that desirous potential customers can discover and then inspect the information on their own, and only if they so choose. In this regard, substantive marketing resembles permission marketing [6].
Being visible helps ensure that the needful, questing prospect that you would never have been able to target on your own is able to see and be aware of your offerings. And, since they are seeking information and answers, your collateral needs to be of a similar nature. Solutions and substance are what they are seeking; what you have run up the flagpole should respond to that.
The mindset here is to respect your prospective customers and to allow them to chose to receive and inspect your offerings, but only if they so choose. If flown in the right venues with the right visibility, customers will see your flags and inspect them if they meet their requirements.
Some of the venues at which you can raise your flags include:
- Blogs — this venue is especially helpful, since you have complete control over content, message, voice and packaging
- Social networks — the value of social networks is now accepted, and should be a core component of any visibility strategy. However, it is also important to make sure that your contributions are driven by substance and value and do not become part of the cacophonous background noise
- Vertical media — there are always existing outlets well-read and -respected by your customer propects. Establishing relationships and value with these third-party outlets can extend your reach
- Web sites — this venue includes your standard Web sites, of course. But, you should also consider setting up specific project-related sites or sites dedicated to documentation (c.f., our TechWiki site of 300+ technical articles) or to methodologies (the excellent MIKE2.0 site is one great example) or to other ways by which particular content (such as tools with the Sweet Tools site) can raise another flag
- User forums — user discussion groups and forums also become their own attractants for like-interested prospects, and
- Conferences and tradeshows — while potentially valuable, presence at conferences and tradeshows must be carefully evaluated. Since participation and opportunity costs are high, the venues should be clearly relevant to your market space with likely decision makers in attendance.
The observant reader will have already concluded that each of these venues develops slowly, and therefore raising visibility is generally a slow-and-steady game that requires patience. Start-up vendors backed by venture firms or those looking for quick visibility and cashout will not find this approach suitable. On the other hand, customer prospects looking for answers and self-sustaining solutions are not much interested in flash in the pan vendors, either.
A Model Responsive to the Changing Nature of Customer Prospects
The real drivers for this changing paradigm come from customer prospects. Sophisticated buyers of enterprise IT and instrumental change agents within organizations share most if not all of these characteristics:
- They are inundated with marketing messages and jaded about hype and “pushed” messages
- They are generally knowledgeable about their needs and problem spaces and about approximate technologies. They are eager and desirous of learning independently and know that their recommendations affect their personal reputations and standing within their enterprises
- With the many volatile external and internal changes, including staff reductions and fluid assignments, leadership for new technology adoption can come from many different and unknown corners of the organization; it is extremely difficult to identify and target prospects
- The economic and competitive environment places a premium on affordability and low-risk evaluations of new technologies
- Lock-ins of any kind — be it to specific vendors or technologies — are understood as inherently risky. This understanding is raising the importance of open and standards-based approaches
- Being the subject of a pushy sales effort is distasteful and a negative to an eventual sale. Education and learning, however, is respected
- Because of all that is at stake, honesty with no bullshit is highly appreciated. If you as a vendor do not offer an appropriate solution or have fulfillment weaknesses, tell the prospect so. Further, tell them who can supply the solution. One never knows when and where the next problem may arise, and providing trustworthy advice can lead to later engagements.
More often than not we find our customers to have already installed and used our existing substantive materials for some time before they approach us about further work. They appreciate the tutorial information and have taught themselves much in advance. By the time we engage, both parties are able to cost-effectively focus on what is truly missing and needed and to deliver those answers in a quick way. Re-engagements tend to occur when a next set of gaps or challenges arise.
Though it may sound trite or even unbelievable to those who have not yet experienced such a relationship, the square deal value offered by substantive marketing can really lead to true partnerships and trust between vendor and customer. We experience it daily with our customers, and vice versa. We also think this is the adaptive approach that our new environment demands.
The Free Path to Open Source and Solutions
Once prospects learn of our substantive offerings, many may decide independently that what we have is not suitable. Others may simply download and use the information on their own, for which we often never know let alone receive revenue. We are completely fine with this, as shown for three different cases.
First, some of these prospects need no more than what we already have. This increases our user base, increases our visibility and often results in contributions to our forums and documentation.
Then, some of these prospects come to learn they need or want more than what our current offerings provide, leading to two possible forks. In one fork, the second case, they may have sufficient skills internally or with other suppliers to extend the system on their own. Some of this flows back to an improved code base or improved installation or documentation bases.
In the other fork, the third case, they may decide to engage us in tailoring a solution for them. That case is the only one of the three that leads to a direct revenue path.
In all three cases we win, and the customer wins. Maybe enterprise software vendors of decades past rue this reality of lower margins and shared benefits; we agree that the absolute profit potential of substantive marketing is much less. But we gladly accept the more enjoyable work and steady revenue relationships resulting from these changes. We are not engaged in some pollyann-ish altruism here, but in a steely-eyed honest brokering that best serves our own self-interest (and fairly that of the customer, as well).
A Square Deal Baseline for Tailored Services
Great IT product does not come from idle musings or dreamed up functionality. It comes solely and directly from solving customer problems. Only via customers can software be refined and made more broadly usable.
A slipstream of those who have previously become aware and tested our offerings will choose to engage our services. This generally takes the form of an inbound call, where the prospect not only qualifies itself, but also establishes the terms and conditions for the sale. They have chosen to select us; they are fish that have jumped into the boat.
To again quote Peter Drucker, “. . . the aim of marketing is to make selling superfluous. The aim of marketing is to know and understand the customer so well that the product or service fits him and sells itself. Ideally, marketing should result in a customer who is ready to buy. All that should be needed then is to make the product or service available . . .” [8]. This is precisely what I meant earlier about the shift in emphasis from sales to marketing.
Even at this point there may be mismatches in needs and our skills and availabilities. If such is the case, we do not hesitate to say so, and attempt to point the prospect in another direction (from which we also gain invaluable market knowledge). If there is indeed a match, we then proceed to try to find common ground on schedule and budget.
Paradoxically, this square deal and honesty about the readiness and weaknesses of our offerings often leads to forgiveness from our customers. For example, for some time we have lacked automated installation scripts that would make it easier for prospects to install our open semantic framework. But, because of compensating value in other areas, such gaps can be overlooked and tackled later on (indeed, as a current customer is now funding). By not pretending to be everything to everyone, we can offer what we do have without embarrassment and get on with the job of solving problems.
For larger potential engagements, we typically suggest a fixed price initial effort to develop an implementation plan. The interviews and research to support this typical 4- to 6-weeks effort (generally in the $5 K to $10 K range, depending) then result in a detailed fulfillment proposal, with firm tasks, budget and schedule, specific to that customer’s requirements. Just as we respect our prospects’ time and budget, we expect the same and do not conduct these detailed plans without compensation. With respect to fulfillment contracts, we cap contract amount and limit milestone payments to pre-set percentages or time expended, whichever is lower.
This approach ensures we understand the customer’s needs and have budgeted and tasked accordingly. Capped contracts also put the onus on us the contractor to understand our own effort and tasking structures and realities, which leads to better future estimating. For the customer, this approach caps risk and potential exposure, and ensures milestones are being met no matter the time expenditures by us, the contractor. This approach extends our square-deal basis to also embrace risks and payments.
New (and Open Source) Developments Fuel the Substance Pipeline
Thus, when customers engage us, they spend almost solely on new functionality specifically tailored to their needs. In doing so, we suggest they agree to release the new developments they fund as open source. We argue — and customers predominantly agree — that they are already benefitting from lower overall costs because other customers have funded sharable, open source before them. We point out that the new customers that follow them will also be independently creating new functionality, to which they will also later benefit.
(This argument does not apply to specific customer data or ontologies, which are naturally proprietary to the customer. Also, if the customer wants to retain intellectual ownership of extensions, we charge higher development fees.)
Once these new developments are completed, they are fed back into a new baseline of valuable content and code. From this new baseline the cycle of substantive marketing can be augmented anew and perpetuated.
Three Guidelines to Leverage Substantive Marketing
All of these points can really be boiled down to three guidelines for how to make substantive marketing effective:
- First, whatever your domain or market, provide useful and substantive content. The content you offer is indeed your marketing collateral. Prospective customers can gauge from it directly whether it meets their needs, appears sound and workable, and has value. If you have little of substance to offer, this paradigm is not for you
- Second, plant many flagpoles and raise your flags high in territories your market prospects are likely to visit. This is a process that requires thoughtfulness and patience. Thoughtfulness, because that is how you determine where to plant your flags. If you yourself are a consumer of what you offer, it is easier to find those venues. And patience, because it takes time to stack valuable content upon valuable content in order to raise visibility
- And, third, be honest and respectful. Help your prospect work within available budget to achieve the most possible at lowest risk. And help them find others, if need be, who might be better able than you to truly solve their problems.
What we are finding — as we continue to refine our understanding of this new paradigm — is that through substantive marketing the fish are finding us and they sometimes jump into the boat. We like our enterprise customers to pre-qualify themselves and already be “sold” once they knock on the door. One never knows when that phone might ring or the email might come in. But when it does, it often results in a collaborative customer as a partner who is a joy to work with to solve exciting new problems.
[2] Paul Graham has been the most prominent observer of this scene; see P. Graham, 2008. “Why There Aren’t Any More Googles,” April 2008 (see
http://www.paulgraham.com/googles.html) and subsequent articles.
[3] See esp. Peter F. Drucker, 1985.
Innovation and Entrepreneurialship: Practice and Principals, Harper & Row, New York, NY, 277 pp.
[4]
Inbound marketing is a marketing strategy that focuses on getting found by customers. According to
David Meerman Scott, inbound marketers “earn their way in” (via publishing helpful information on a blog etc.) in contrast to outbound marketing where they used to have to “buy, beg, or bug their way in” (via paid advertisements, issuing press releases in the hope they get picked up by the trade press, or paying commissioned sales people, respectively).
Brian Halligan, cofounder and CEO of
HubSpot, claims he first coined the term of inbound marketing.
[5]
Content marketing is an umbrella term encompassing all marketing formats that involve the creation or sharing of content for the purpose of engaging current and potential consumer bases. In contrast to traditional marketing methods that aim to increase sales or awareness through interruption techniques, content marketing subscribes to the notion that delivering high-quality, relevant and valuable information to prospects and customers drives profitable consumer action. See also Holger Shulze, 2011.
B2B Content Marketing Trends slideshow, see
http://www.slideshare.net/hschulze/b2b-content-marketing-report.
[6]
Seth Godin coined the term
permission marketing wherein marketers obtain permission before advancing to the next step in the purchasing process. It is mostly used by online marketers, notably email marketers and search marketers, as well as certain direct marketers who send a catalog in response to a request. Godin contrasts this approach to traditional “interruption marketing” where messages are sent without prior permission.
[7] See the three-part series, M.K. Bergman, 2010. “Listening to the Enterprise: Total Open Solutions,” “
Part 1,” “
Part 2” and “
Part 3,”
AI3:::Adaptive Information blog, May 12 – 31, 2010.
[8] Peter F. Drucker, 1974.
Management: Tasks, Responsibilities, Practices. New York, NY: Harper & Row. pp. 864. ISBN 0-06-011092-9.
[9] The intro photo is of the world’s tallest flagpole (at 165 m), in Dushanbe, Tajikistan. The photo is courtesy of
CentralAsiaOnline.com.