Author’s Note: This is an on line version of a paper that Mike Bergman recently released under the auspices of BrightPlanet Corp The citation for this effort is:
M.K. Bergman, “Tutorial: Internet Languages, Character Sets and Encodings,” BrightPlanet Corporation Technical Documentation, March 2006, 13 pp.
Click here to obtain a PDF copy of this posting (13 pp, 79 K)
Broad-scale, international open source harvesting from the Internet poses many challenges in use and translation of legacy encodings that have vexed academics and researchers for many years. Successfully addressing these challenges will only grow in importance as the relative percentage of international sites grows in relation to conventional English ones.
A major challenge in internationalization and foreign source support is “encoding.” Encodings specify the arbitrary assignment of numbers to the symbols (characters or ideograms) of the world’s written languages needed for electronic transfer and manipulation. One of the first encodings developed in the 1960s was ASCII (numerals, plus a-z; A-Z); others developed over time to deal with other unique characters and the many symbols of (particularly) the Asiatic languages.
Some languages have many character encodings and some encodings, for example Chinese and Japanese, have very complex systems for handling the large number of unique characters. Two different encodings can be incompatible by assigning the same number to two distinct symbols, or vice versa. So-called Unicode set out to consolidate many different encodings, all using separate code plans into a single system that could represent all written languages within the same character encoding. There are a few Unicode techniques and formats, the most common being UTF-8.
The Internet was originally developed via efforts in the United States funded by ARPA (later DARPA) and NSF, extending back to the 1960s. At the time of its commercial adoption in the early 1990s via the Word Wide Web protocols, it was almost entirely dominated by English by virtue of this U.S. heritage and the emergence of English as the lingua franca of the technical and research community.
However, with the maturation of the Internet as a global information repository and means for instantaneous e-commerce, today’s online community now approaches 1 billion users from all existing countries. The Internet has become increasingly multi-lingual.
Efficient and automated means to discover, search, query, retrieve and harvest content from across the Internet thus require an understanding of the source human languages in use and the means to encode them for electronic transfer and manipulation. This Tutorial provides a brief introduction to these topics.
Internet Language Use
Yoshiki Mikami, who runs the UN’s Language Observatory, has an interesting way to summarize the languages of the world. His updated figures, plus some other BrightPlanet statistics are:[1]
Category
|
Number
|
Source or Notes
|
Active Human Languages |
6,912
|
from www.ethnologue.com |
Language Identifiers |
440
|
based on ISO 639 |
Human Rights Translation |
327
|
UN’s Universal Declaration of Human Rights (UDHR) |
Unicode Languages |
244
|
see text |
DQM Languages |
140
|
estimate based on prevalence, BT input |
Windows XP Languages |
123
|
from Microsoft |
Basis Tech Languages |
40
|
based on Basis Tech’s Rosette Language Identifier (RLI) |
Google Search Languages |
35
|
from Google |
There are nearly 7,000 living languages spoken today, though most have few speakers and many are becoming extinct. About 347 (or approximately 5%) of the world’s languages have at least one million speakers and account for 94% of the world’s population. Of this amount, 83 languages account for 80% of the world’s population, with just 8 languages with greater than 100 million speakers accounting for about 40% of total population. By contrast, the remaining 95% of languages are spoken by only 6% of the world’s people.[2]
This prevalence is shown by the fact that the UN’s Universal Declaration of Human Rights (UDHR) has only been translated into those languages generally with 1 million or more speakers.
The remaining items on the table above enumerate languages that can be represented electronically, or are “encoded.” More on this topic is provided below.
Of course, native language does not necessarily equate to Internet use, with English predominating because of multi-lingualism, plus the fact that richer countries or users within countries exhibit greater Internet access and use.
The most recent comprehensive figures for Internet language use and prevalence are from the Global Reach Web site for late 2004, with only percentage figures shown for ease of reading for those countries with greater than a 1.0% value:[3] [4]
|
Percent of
|
2003 Internet Users
|
Global Population
|
|
Web Pages
|
Millions
|
Percent
|
Millions
|
Percent
|
|
|
|
|
|
|
ENGLISH |
68.4%
|
287.5
|
35.6%
|
508
|
8.0%
|
NON-ENGLISH |
31.6%
|
519.6
|
64.4%
|
5,822
|
92.0%
|
|
|
|
|
|
|
EUROPEAN (non-English) |
|
|
|
|
|
Catalan |
|
2.9
|
|
7
|
|
Czech |
|
4.2
|
|
12
|
|
Dutch |
|
13.5
|
1.7%
|
20
|
|
Finnish |
|
2.8
|
|
6
|
|
French |
3.0%
|
28.0
|
3.5%
|
77
|
1.2%
|
German |
5.8%
|
52.9
|
6.6%
|
100
|
1.6%
|
Greek |
|
2.7
|
|
12
|
|
Hungarian |
|
1.7
|
|
10
|
|
Italian |
1.6%
|
24.3
|
3.0%
|
62
|
1.0%
|
Polish |
|
9.5
|
1.2%
|
44
|
|
Portuguese |
1.4%
|
25.7
|
3.2%
|
176
|
2.8%
|
Romanian |
|
2.4
|
|
26
|
|
Russian |
1.9%
|
18.5
|
2.3%
|
167
|
2.6%
|
Scandinavian |
|
14.6
|
1.8%
|
20
|
|
Danish |
|
3.5
|
|
5
|
|
Icelandic |
|
0.2
|
|
0
|
|
Norwegian |
|
2.9
|
|
5
|
|
Swedish |
|
7.9
|
1.0%
|
9
|
|
Serbo-Croatian |
|
1.0
|
|
20
|
|
Slovak |
|
1.2
|
|
6
|
|
Slovenian |
|
0.8
|
|
2
|
|
Spanish |
2.4%
|
65.6
|
8.1%
|
350
|
5.5%
|
Turkish |
|
5.8
|
|
67
|
1.1%
|
Ukrainian |
|
0.9
|
|
47
|
|
SUB-TOTAL |
18.7%
|
279.0
|
34.6%
|
1,230
|
19.4%
|
|
|
|
|
|
|
ASIAN LANGUAGES |
|
|
|
|
|
Arabic |
|
10.5
|
1.3%
|
300
|
4.7%
|
Chinese |
3.9%
|
102.6
|
12.7%
|
874
|
13.8%
|
Farsi |
|
3.4
|
|
64
|
1.0%
|
Hebrew |
|
3.8
|
|
5
|
|
Japanese |
5.9%
|
69.7
|
8.6%
|
125
|
2.0%
|
Korean |
1.3%
|
29.9
|
3.7%
|
78
|
1.2%
|
Malay |
|
13.6
|
1.7%
|
229
|
3.6%
|
Thai |
|
4.9
|
|
46
|
|
Vietnamese |
|
2.2
|
|
68
|
1.1%
|
SUB-TOTAL |
12.9%
|
240.6
|
29.8%
|
1,789
|
28.3%
|
|
|
|
|
|
|
TOTAL WORLD |
100.0%
|
807.1
|
100.0%
|
6,330
|
100.0%
|
English speakers have nearly a five-fold increase in Internet use than sheer population would suggest, and about an eight-fold increase in percent of English Web pages. However, various census efforts over time have shown a steady decrease in this English prevalence (data not shown.)
Virtually all European languages show higher Internet prevalence than actual population would suggest; Asian languages show the opposite. (African languages are even less represented than population would suggest; data not shown.)
Internet penetration appears to be about 20% of global population and growing rapidly. It is not unlikely that percentages of Web users and the pages the Web is written in will continue to converge to real population percentages. Thus, over time and likely within the foreseeable future, users and pages should more closely approximate the percentage figures shown in the rightmost column in the table above.
Script Families
Another useful starting point for understanding languages and their relation to the Internet is a 2005 UN publication from a World Summit on the Information Society. This 113 pp. report can be found at http://www.uis.unesco.org/template/pdf/cscl/MeasuringLinguisticDiversity_En.pdf.[5]
Languages have both a representational form and meaning. The representational form is captured by scripts, fonts or ideograms. The meaning is captured by semantics. In an electronic medium, it is the representational form that must be transmitted accurately. Without accurate transmittal of the form, it is impossible to manipulate that language or understand its meaning.
Representational forms fit within what might be termed script families. Script families are not strictly alphabets or even exact character or symbol matches. They represent similar written approaches and some shared characteristics.
For example, English and its German and Romance language cousins share very similar, but not identical, alphabets. Similarly, the so-called CJK (Chinese, Japanese, Korean) share a similar approach to using ideograms without white space between tokens or punctuation.
At the highest level, the world’s languages may be clustered into these following script families:[6]
Script
|
Latin
|
Cyrillic
|
Arabic
|
Hanzi
|
Indic
|
Others*
|
Million users |
2,238
|
451
|
462
|
1,085
|
807
|
129
|
% of Total |
43.3%
|
8.7%
|
8.9%
|
21.0%
|
15.6%
|
2.5%
|
Key languages |
Romance (European) Slavic (some) Vietnamese Malay Indonesian |
Russian Slavic (some) Kazakh Uzbek |
Arabic Urdu Persian Pashtu |
Chinese Japanese Korean |
Hindi Tamil Bengali Punjabi Sanskrit Thai |
Greek Hebrew Georgian Assyrian Armenian |
Note that English and the Romance languages fall within the Latin script family, the CJK within Hanzi. The “Other” category is a large catch-all, including Greek, Hebrew, many African languages, and others. However, besides Greek and Hebrew, most specific languages of global importance are included in the other named families. Also note that due to differences in sources, that total user counts do not equal earlier tables.
Character Sets and Encodings
In order to take advantage of the computer’s ability to manipulate text (e.g., displaying, editing, sorting, searching and efficiently transmitting it), communications in a given language needs to be represented in some kind of encoding. Encodings specify the arbitrary assignment of numbers to the symbols of the world’s written languages. Two different encodings can be incompatible by assigning the same number to two distinct symbols, or vice versa. Thus, much of what the Internet offers with respect to linguistic diversity comes down to the encodings available for text.
The most widely used encoding is the American Standard Code for Information Interchange (ASCII), a code devised during the 1950s and 1960s under the auspices of the American National Standards Institute (ANSI) to standardize teletype technology. This encoding comprises 128 character assignments (7-bit) and is suitable primarily for North American English.[6]
Historically, other languages that did not fit in the ASCII 7-bit character set (a-z; A-Z) pretty much created their own character sets, sometimes with local standards acceptance and sometimes not. Some languages have many character encodings and some encodings, particularly Chinese and Japanese, have very complex systems for handling the large number of unique characters. Another difficult group is Hindi and the Indic language family, with speakers that number into the hundreds of millions. According to one University of Southern California researcher, almost every Hindi language web site has its own encoding.[7]
The Internet Assigned Names and Authority (IANA) organization maintains a master list of about 245 standard charset (“character set”) encodings and 550 associated aliases to the same used in one manner or another on the Internet.[8] [9] Some of these electronic encodings were created by large vendors with a stake in electronic transfer such as IBM, Microsoft, Apple and the like. Other standards result from recognized standards organizations such as ANSI, ISO, Unicode and the like. Many of these standards date back as far as the 1960s; many others are specific to certain countries.
Earlier estimates showed on the range of 40 to 250 languages per named encoding type. While no known estimate exists, if one assumes 100 languages for each of the IANA-listed encodings, there could be on the order of 25,000 or so specific language-encoding combinations possible on the Internet based on these “standards.” There are perhaps thousands of specific language encodings also extant.
Whatever the numbers, clearly it is critical to identify accurately the specific encoding and its associated language for any given Web page or database site. Without this accuracy, it is impossible to electronically query and understand the content.
As might be suspected, this topic too is very broad. For a very comprehensive starting point on all topics related to encodings and character sets, please see I18N (which stands for “internationalization”) Guy’s Web site at http://www.i18nguy.com/unicode/codepages.html.
Unicode
In the late 1980s, there were two independent attempts to create a single unified character set. One was the ISO 10646 project of the International Organization for Standardization (ISO), the other was the Unicode Project organized by a consortium of (initially mostly US) manufacturers of multi-lingual software. Fortunately, the participants of both projects realized in 1991 that two different unified character sets did not make sense and they joined efforts to create a single code table, now referred to as Unicode. While both projects still exist and publish their respective standards independently, the Unicode Consortium and ISO/IEC JTC1/SC2 have agreed to keep the code tables of the Unicode and ISO 10646 standards compatible and closely coordinated.
Unicode sets out to consolidate many different encodings, all using separate code plans into a single system that can represent all written languages within the same character encoding. Unicode is first a set of code tables to assign integer numbers to characters, also called a code point. Unicode then has several methods for how a sequence of such characters or their respective integer values can be represented as a sequence of bytes, generally prefixed by “UTF.”
In UTF-8, the most common method, every code point from 0-127 is stored in a single byte. Only code points 128 and above are stored using 2, 3 or up to 6 bytes. This method has the advantage that English text looks exactly the same in UTF-8 as it did in ASCII, so ASCII is a conforming sub-set. More unusual characters such as accented letters, Greek letters or CJK ideograms may need several bytes to store a single code point.
The traditional store-it-in-two-byte method for Unicode is called UCS-2 (because it has two bytes) or UTF-16 (because it has 16 bits). There’s something called UTF-7, which is a lot like UTF-8 but guarantees that the high bit will always be zero. There’s UTF-4, which stores each code point in 4 bytes, which has the nice property that every single code point can be stored in the same number of bytes. There is also UTF-32 that stores the code point in 32 bits but requires more storage. Regardless, UTF-7, -8, -16, and -32 all have the property of being able to store any code point correctly.
BrightPlanet, along with many others, has adopted UTF-8 as the standard Unicode method to process all string data. There are tools available to convert nearly any existing character encoding into a UTF-8 encoded string. Java supplies these tools as does Basis Technolgy, one of BrightPlanet’s partners in language processing.
As presently defined, Unicode supports about 245 common languages according to a variety of scripts (see notes at end of the table):[10]
Language
|
Script(s)
|
Some Country Notes
|
Abaza |
Cyrillic |
|
Abkhaz |
Cyrillic |
|
Adygei |
Cyrillic |
|
Afrikaans |
Latin |
|
Ainu |
Katakana, Latin |
Japan |
Aisor |
Cyrillic |
|
Albanian |
Latin [2] |
|
Altai |
Cyrillic |
|
Amharic |
Ethiopic |
Ethiopia |
Amo |
Latin |
Nigeria |
Arabic |
Arabic |
|
Armenian |
Armenian, Syriac [3] |
|
Assamese |
Bengali |
Bangladesh, India |
Assyrian (modern) |
Syriac |
|
Avar |
Cyrillic |
|
Awadhi |
Devanagari |
India, Nepal |
Aymara |
Latin |
Peru |
Azeri |
Cyrillic, Latin |
|
Azerbaijani |
Arabic, Cyrillic, Latin |
|
Badaga |
Tamil |
India |
Bagheli |
Devanagari |
India, Nepal |
Balear |
Latin |
|
Balkar |
Cyrillic |
|
Balti |
Devanagari, Balti [2] |
India, Pakistan |
Bashkir |
Cyrillic |
|
Basque |
Latin |
|
Batak |
Batak [1], Latin |
Philippines, Indonesia |
Batak toba |
Batak [1], Latin |
Indonesia |
Bateri |
Devanagari |
(aka Bhatneri) India, Pakistan |
Belarusian |
Cyrillic |
(aka Belorussian, Belarusan) |
Bengali |
Bengali |
Bangladesh, India |
Bhili |
Devanagari |
India |
Bhojpuri |
Devanagari |
India |
Bihari |
Devanagari |
India |
Bosnian |
Latin |
Bosnia-Herzegovina |
Braj bhasha |
Devanagari |
India |
Breton |
Latin |
France |
Bugis |
Buginese [1] |
Indonesia, Malaysia |
Buhid |
Buhid |
Philippines |
Bulgarian |
Cyrillic |
|
Burmese |
Myanmar |
|
Buryat |
Cyrillic |
|
Bahasa |
Latin |
(see Indonesian) |
Catalan |
Latin |
|
Chakma |
Bengali, Chakma [1] |
Bangladesh, India |
Cham |
Cham [1] |
Cambodia, Thailand, Viet Nam |
Chechen |
Cyrillic |
Georgia |
Cherokee |
Cherokee, Latin |
|
Chhattisgarhi |
Devanagari |
India |
Chinese |
Han |
|
Chukchi |
Cyrillic |
|
Chuvash |
Cyrillic |
|
Coptic |
Greek |
Egypt |
Cornish |
Latin |
United Kingdom |
Corsican |
Latin |
|
Cree |
Canadian Aboriginal Syllabics, Latin |
|
Croatian |
Latin |
|
Czech |
Latin |
|
Danish |
Latin |
|
Dargwa |
Cyrillic |
|
Dhivehi |
Thaana |
Maldives |
Dungan |
Cyrillic |
|
Dutch |
Latin |
|
Dzongkha |
Tibetan |
Bhutan |
Edo |
Latin |
|
English |
Latin, Deseret [3], Shavian [3] |
|
Esperanto |
Latin |
|
Estonian |
Latin |
|
Evenki |
Cyrillic |
|
Faroese |
Latin |
Faroe Islands |
Farsi |
Arabic |
(aka Persian) |
Fijian |
Latin |
|
Finnish |
Latin |
|
French |
Latin |
|
Frisian |
Latin |
|
Gaelic |
Latin |
|
Gagauz |
Cyrillic |
|
Garhwali |
Devanagari |
India |
Garo |
Bengali |
Bangladesh, India |
Gascon |
Latin |
|
Ge’ez |
Ethiopic |
Eritrea, Ethiopia |
Georgian |
Georgian |
|
German |
Latin |
|
Gondi |
Devanagari, Telugu |
India |
Greek |
Greek |
|
Guarani |
Latin |
|
Gujarati |
Gujarati |
|
Garshuni |
Syriac |
|
Hanunóo |
Latin, Hanunóo |
Philippines |
Harauti |
Devanagari |
India |
Hausa |
Latin, Arabic [3] |
|
Hawaiian |
Latin |
|
Hebrew |
Hebrew |
|
Hindi |
Devanagari |
|
Hmong |
Latin, Hmong [1] |
|
Ho |
Devanagari |
Bangladesh, India |
Hopi |
Latin |
|
Hungarian |
Latin |
|
Ibibio |
Latin |
|
Icelandic |
Latin |
|
Indonesian |
Arabic [3], Latin |
|
Ingush |
Arabic, Latin |
|
Inuktitut |
Canadian Aboriginal Syllabics, Latin |
Canada |
Iñupiaq |
Latin |
Greenland |
Irish |
Latin |
|
Italian |
Latin |
|
Japanese |
Han + Hiragana + Katakana |
|
Javanese |
Latin, Javanese [1] |
|
Judezmo |
Hebrew |
|
Kabardian |
Cyrillic |
|
Kachchi |
Devanagari |
India |
Kalmyk |
Cyrillic |
|
Kanauji |
Devanagari |
India |
Kankan |
Devanagari |
India |
Kannada |
Kannada |
India |
Kanuri |
Latin |
|
Khanty |
Cyrillic |
|
Karachay |
Cyrillic |
|
Karakalpak |
Cyrillic |
|
Karelian |
Latin, Cyrillic |
|
Kashmiri |
Devanagari, Arabic |
|
Kazakh |
Cyrillic |
|
Khakass |
Cyrillic |
|
Khamti |
Myanmar |
India, Myanmar |
Khasi |
Latin, Bengali |
Bangladesh, India |
Khmer |
Khmer |
Cambodia |
Kirghiz |
Arabic [3], Latin, Cyrillic |
|
Komi |
Cyrillic, Latin |
|
Konkan |
Devanagari |
|
Korean |
Hangul + Han |
|
Koryak |
Cyrillic |
|
Kurdish |
Arabic, Cyrillic, Latin |
Iran, Iraq |
Kuy |
Thai |
Cambodia, Laos, Thailand |
Ladino |
Hebrew |
|
Lak |
Cyrillic |
|
Lambadi |
Telugu |
India |
Lao |
Lao |
Laos |
Lapp |
Latin |
(see Sami) |
Latin |
Latin |
|
Latvian |
Latin |
|
Lawa, eastern |
Thai |
Thailand |
Lawa, western |
Thai |
China, Thailand |
Lepcha |
Lepcha [1] |
Bhutan, India, Nepal |
Lezghian |
Cyrillic |
|
Limbu |
Devanagari, Limbu [1] |
Bhutan, India, Nepal |
Lisu |
Lisu (Fraser) [1], Latin |
China |
Lithuanian |
Latin |
|
Lushootseed |
Latin |
USA |
Luxemburgish |
Latin |
(aka Luxembourgeois) |
Macedonian |
Cyrillic |
|
Malay |
Arabic [3], Latin |
Brunei, Indonesia, Malaysia |
Malayalam |
Malayalam |
|
Maldivian |
Thaana |
Maldives (See Dhivehi) |
Maltese |
Latin |
|
Manchu |
Mongolian |
China |
Mansi |
Cyrillic |
|
Marathi |
Devanagari |
India |
Mari |
Cyrillic, Latin |
|
Marwari |
Devanagari |
|
Meitei |
Meetai Mayek [1], Bengali |
Bangladesh, India |
Moldavian |
Cyrillic |
|
Mon |
Myanmar |
Myanmar, Thailand |
Mongolian |
Mongolian, Cyrillic |
China, Mongolia |
Mordvin |
Cyrillic |
|
Mundari |
Bengali, Devanagari |
Bangladesh, India, Nepal |
Naga |
Latin, Bengali |
India |
Nanai |
Cyrillic |
|
Navajo |
Latin |
|
Naxi |
Naxi [2] |
China |
Nenets |
Cyrillic |
|
Nepali |
Devanagari |
|
Netets |
Cyrillic |
|
Newari |
Devanagari, Ranjana, Parachalit |
|
Nogai |
Cyrillic |
|
Norwegian |
Latin |
|
Oriya |
Oriya |
Bangladesh, India |
Oromo |
Ethiopic |
Egypt, Ethiopia, Somalia |
Ossetic |
Cyrillic |
|
Pali |
Sinhala, Devanagari, Thai |
India, Myanmar, Sri Lanka |
Panjabi |
Gurmukhi |
India (see Punjabi) |
Parsi-dari |
Arabic |
Afghanistan, Iran |
Pashto |
Arabic |
Afghanistan |
Polish |
Latin |
|
Portuguese |
Latin |
|
Provençal |
Latin |
|
Prussian |
Latin |
|
Punjabi |
Gurmukhi |
India |
Quechua |
Latin |
|
Riang |
Bengali |
Bangladesh, China, India, Myanmar |
Romanian |
Latin, Cyrillic [3] |
(aka Rumanian) |
Romany |
Cyrillic, Latin |
|
Russian |
Cyrillic |
|
Sami |
Cyrillic, Latin |
|
Samaritan |
Hebrew, Samaritan [1] |
Israel |
Sanskrit |
Sinhala, Devanagari, etc. |
India |
Santali |
Devanagari, Bengali, Oriya, Ol Cemet [1] |
India |
Selkup |
Cyrillic |
|
Serbian |
Cyrillic |
|
Shan |
Myanmar |
China, Myanmar, Thailand |
Sherpa |
Devanagari |
|
Shona |
Latin |
|
Shor |
Cyrillic |
|
Sindhi |
Arabic |
|
Sinhala |
Sinhala |
(aka Sinhalese) Sri Lanka |
Slovak |
Latin |
|
Slovenian |
Latin |
|
Somali |
Latin |
|
Spanish |
Latin |
|
Swahili |
Latin |
|
Swedish |
Latin |
|
Sylhetti |
Siloti Nagri [1], Bengali |
Bangladesh |
Syriac |
Syriac |
|
Swadaya |
Syriac |
(see Syriac) |
Tabasaran |
Cyrillic |
|
Tagalog |
Latin, Tagalog |
|
Tagbanwa |
Latin, Tagbanwa |
|
Tahitian |
Latin |
|
Tajik |
Arabic [3], Latin, Cyrillic (? Latin) |
(aka Tadzhik) |
Tamazight |
Tifinagh [1], Latin |
|
Tamil |
Tamil |
|
Tat |
Cyrillic |
|
Tatar |
Cyrillic |
|
Telugu |
Telugu |
|
Thai |
Thai |
|
Tibetan |
Tibetan |
|
Tigre |
Ethiopic |
Eritrea, Sudan |
Tsalagi |
(see Cherokee) |
|
Tulu |
Kannada |
India |
Turkish |
Arabic [3], Latin |
|
Turkmen |
Arabic [3], Latin, Cyrillic (? Latin) |
|
Tuva |
Cyrillic |
|
Turoyo |
Syriac |
(see Syriac) |
Udekhe |
Cyrillic |
|
Udmurt |
Cyrillic, Latin |
|
Uighur |
Arabic, Latin, Cyrillic, Uighur [1] |
|
Ukranian |
Cyrillic |
|
Urdu |
Arabic |
|
Uzbek |
Cyrillic, Latin |
|
Valencian |
Latin |
|
Vietnamese |
Latin, Chu Nom |
|
Yakut |
Cyrillic |
|
Yi |
Yi, Latin |
|
Yiddish |
Hebrew |
|
Yoruba |
Latin |
|
|
|
|
[1] = Not yet encoded in Unicode. |
|
[2] = Has one or more extinct or minor native script(s), not yet encoded. |
[3] = Formerly or historically used this script, now uses another. |
Notice most of these scripts fall into the seven broader script families such as Latin, Hanzi and Indic noted previously.
While more countries are adopting Unicode and sample results indicate increasing percentage use, it is by no means prevalent. In general, Europe has been slow to embrace Unicode with many legacy encodings still in use, perhaps Arabic sites have reached the 50% level, and Asian use is problematic.[11] Other samples suggest that UTF-8 encoding is limited to 8.35% of all Asian Web pages. Some countries, such as Nepal, Vietnam and Tajikistan exceed 70% compliance, while others such Syria, Laos and Brunei are below even 1%.[12] According to the Archive Pass project, which also used Basis Tech’s RLI for encoding detection, Chinese sites are dominated by GB-2312 and Big 5 encodings, while Shift-JIS is most common for Japanese.[13]
Detecting and Communicating with Legacy Encodings
There are two primary problems when dealing with non-Unicode encodings; identifying what the encoding is and converting that encoding to a Unicode string, usually UTF-8. Detecting the encoding is a difficult process, BasisTech’s RLI does an excellent job. Converting the non-Unicode string to a Unicode string can be easily done using tools available in the Java JDK, or using BasisTech’s RCLU library.
Basis Tech detects a combination of 96 language encoding pairs involving 40 different languages and 30 unique encoding types:
Language
|
Encoding
|
Albanian |
UTF-8, Windows-1252 |
Arabic |
UTF-8, Windows-1256, ISO-8859-6 |
Bahasa Indonesia |
UTF-8, Windows-1252 |
Bahasa Malay |
UTF-8, Windows-1252 |
Bulgarian |
UTF-8, Windows-1251, ISO-8859-5, KOI8-R |
Catalan |
UTF-8, Windows-1252 |
Chinese |
UTF-8, GB-2312, HZ-GB-2312, ISO-2022-CN |
Chinese |
UTF-8, Big5 |
Croatian |
UTF-8, Windows-1250 |
Czech |
UTF-8, Windows-1250 |
Danish |
UTF-8, Windows-1252 |
Dutch |
UTF-8, Windows-1252 |
English |
UTF-8, Windows-1252 |
Estonian |
UTF-8, Windows-1257 |
Farsi |
UTF-8, Windows-1256 |
Finnish |
UTF-8, Windows-1252 |
French |
UTF-8, Windows-1252 |
German |
UTF-8, Windows-1252 |
Greek |
UTF-8, Windows-1253 |
Hebrew |
UTF-8, Windows-1255 |
Hungarian |
UTF-8, Windows-1250 |
Icelandic |
UTF-8, Windows-1252 |
Italian |
UTF-8, Windows-1252 |
Japanese |
UTF-8, EUC-JP, ISO-2022-JP, Shift-JIS |
Korean |
UTF-8, EUC-KR, ISO-2022-KR |
Latvian |
UTF-8, Windows-1257 |
Lithuanian |
UTF-8, Windows-1257 |
Norwegian |
UTF-8, Windows-1252 |
Polish |
UTF-8, Windows-1250 |
Portuguese |
UTF-8, Windows-1252 |
Romanian |
UTF-8, Windows-1250 |
Russian |
UTF-8, Windows-1251, ISO-8859-5, IBM-866, KOI8-R, x-Mac-Cyrillic |
Slovak |
UTF-8, Windows-1250 |
Slovenian |
UTF-8, Windows-1250 |
Spanish |
UTF-8, Windows-1252 |
Swedish |
UTF-8, Windows-1252 |
Tagalog |
UTF-8, Windows-1252 |
Thai |
UTF-8, Windows-874 |
Turkish |
UTF-8, Windows-1254 |
Vietnamese |
UTF-8, VISCII, VPS, VIQR, TCVN, VNI |
Java SDK encoding/decoding supports 22 basic European, and 125 other international forms (mostly non-European), for 147 total. If an ecoded form is not on this list, and not already Unicode, software can not talk to the site without special converters or adapters. See http://java.sun.com/j2se/1.5.0/docs/guide/intl/encoding.doc.html
Of course, to avoid the classic “garbage in, garbage out” (GIGO) problem, accurate detection must be made of the source’s encoding type, there must be a converter for that type into a canonical, internal form (such as UTF-8), and another converter must exist for converting that canonical form back to the source’s original encoding. The combination of the existing Basis Tech RLI and the Java SDK produce a valid combination of 89 language/encoding pairs (with invalid combinations shown in Bold Red above.)
Fortunately, existing valid combinations appear to cover all prevalent languages and encoding types. Should gaps exist, specialized detectors and converters may be required. As events move forward, the family of Indic languages may be the most problematic for expansion with standard tools.
Actual Language Processing
Encoding detection, and the resulting proper storage and language identification, is but the first essential step in actual language processing. Additional tools in morphological analysis or machine translation may need to be applied to address actual analyst needs. These tools are beyond the scope of this Tutorial.
The key point, however, is that all foreign language processing and analysis begins with accurate encoding detection and communicating with the host site in its original encoding. These steps are the sine qua non of language processing.
Exemplar Methodology for Internet Foreign Language Support
We can now take the information in this Tutorial and present what might be termed an exemplar methodology for initial language detection and processing. A schematic of this methodology is provided in the following diagram:
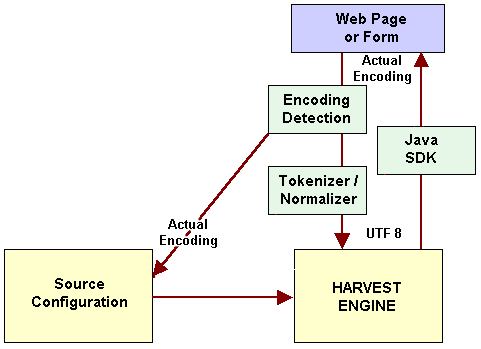
This diagram shows that the actual encoding for an original Web document or search form must be detected, converted into a standard “canonical” form for internal storage, but talked to in its actual native encoding form when searching it. Encoding detection software and utilities within the Java SDK can aid this process greatly.
And, as the proliferation of languages and legacy forms grows, we can expect such utilities to embrace an ever-widening set of encodings.
[1] Yoshiki Mikami, “Language Observatory: Scanning Cyberspace for Languages,” from The Second Language Observatory Workshop, February 21-25, 2005, 41 pp. See http://gii.nagaokaut.ac.jp/~zaidi/Proceedings%20Online/01_Mikami.pdf. This is a generally useful reference on Internet and language. Please note some of the figures have been updated with more recent data.
[2] See http://www.ethnologue.com/ethno_docs/distribution.asp?by=size.
[3] See http://global-reach.biz/globstats/index.php3. Also, for useful specific notes by country as well as orignial references, see http://global-reach.biz/globstats/refs.php3.
[4] Another interesting language source with an emphasis on Latin family langguages is FUNREDES’ 2005 study of languages and cultures. See http://funredes.org/LC/english/index.html.
[5] John Paolillo, Daniel Pimienta, Daniel Prado, et al. Measuring Linguistic Diversity on the Internet, a UNESCO Publications for the World Summit on the Information Society 2005, 113 pp. See http://www.uis.unesco.org/template/pdf/cscl/MeasuringLinguisticDiversity_En.pdf
[6] John Paolillo, “Language Diversity on the Internet,” pp. 43-89, in John Paolillo, Daniel Pimienta, Daniel Prado, et al., Measuring Linguistic Diversity on the Internet, UNESCO Publications for the World Summit on the Information Society 2005, 113 pp. See http://www.uis.unesco.org/template/pdf/cscl/MeasuringLinguisticDiversity_En.pdf.
[7] Information Sciences Institute press release, “USC Researchers Build Machine Translation System — and More — for Hindi in Less Than a Month,” June 30, 2003. See http://www.isi.edu/stories/60.html.
[8] http://www.iana.org/assignments/character-sets.
[9] The actual values were calculated from Jukka “Yucca” Korpela’s informative Web site at http://www.cs.tut.fi/%7Ejkorpela/chars/sorted.html.
[10] See http://www.unicode.org/onlinedat/languages-scripts.html.
[11] Pers. Comm., B. Margulies, Basis Technology, Inc., Feb. 27, 2006.
[12] Yoshika Mikami et al., “Language Diversity on the Internet: An Asian View,” pp. 91-103, in John Paolillo, Daniel Pimienta, Daniel Prado, et al., Measuring Linguistic Diversity on the Internet, UNESCO Publications for the World Summit on the Information Society 2005, 113 pp. See http://www.uis.unesco.org/template/pdf/cscl/MeasuringLinguisticDiversity_En.pdf.
[13] Archive Pass Project; see http://crawler.archive.org/cgi-bin/wiki.pl?ArchivePassProject